Note
Click here to download the full example code
Compares implementations of Add#
This example compares the addition of numpy to onnxruntime implementation. Function numpy.add is repeated 3 times. This minimizes the cost of copying the data from python to an external library. If available, tensorflow and pytorch are included as well. The numpy implementation is not the best, it allocates more buffers than necessary because parameter out is not used to reuse buffers.
import numpy
import pandas
import matplotlib.pyplot as plt
from onnxruntime import InferenceSession
from skl2onnx.common.data_types import FloatTensorType
from skl2onnx.algebra.onnx_ops import OnnxAdd
from cpyquickhelper.numbers import measure_time
from tqdm import tqdm
from mlprodict.testing.experimental_c_impl.experimental_c import code_optimisation
print(code_optimisation())
Out:
AVX-omp=8
Add implementations#
try:
from tensorflow.math import add as tf_add
from tensorflow import convert_to_tensor
except ImportError:
tf_add = None
try:
from torch import add as torch_add, from_numpy
except ImportError:
torch_add = None
def build_ort_add(op_version=12):
node1 = OnnxAdd('x', 'y', op_version=op_version)
node2 = OnnxAdd(node1, 'y', op_version=op_version)
node = OnnxAdd(node2, 'y', op_version=op_version, output_names=['z'])
onx = node.to_onnx(inputs=[('x', FloatTensorType()),
('y', FloatTensorType())],
target_opset=op_version)
sess = InferenceSession(onx.SerializeToString())
return lambda x, y: sess.run(None, {'x': x, 'y': y})
def loop_fct(fct, xs, ys):
for x, y in zip(xs, ys):
fct(x, y)
def benchmark_op(repeat=5, number=2, name="Add", shape_fcts=None):
if shape_fcts is None:
def shape_fct(dim):
return (5, dim, dim)
shape_fcts = (shape_fct, shape_fct)
ort_fct = build_ort_add()
res = []
for dim in tqdm([8, 16, 32, 64, 100, 128, 200,
256, 400, 512, 1024, 1536, 2048, 2560]):
shape1 = shape_fcts[0](dim)
shape2 = shape_fcts[1](dim)
n_arrays = (16 if dim < 512 else 4) if dim < 2048 else 4
if len(shape1) > 3:
n_arrays = int(n_arrays / 4)
xs = [numpy.random.rand(*shape1).astype(numpy.float32)
for _ in range(n_arrays)]
ys = [numpy.random.rand(*shape2).astype(numpy.float32)
for _ in range(n_arrays)]
info = dict(shape1=shape1, shape2=shape2)
# numpy
ctx = dict(
xs=xs, ys=ys,
fct=lambda x, y: numpy.add(numpy.add(numpy.add(x, y), y), y),
loop_fct=loop_fct)
obs = measure_time(
"loop_fct(fct, xs, ys)",
div_by_number=True, context=ctx, repeat=repeat, number=number)
obs['dim'] = dim
obs['fct'] = 'numpy'
obs.update(info)
res.append(obs)
# onnxruntime
ctx['fct'] = ort_fct
obs = measure_time(
"loop_fct(fct, xs, ys)",
div_by_number=True, context=ctx, repeat=repeat, number=number)
obs['dim'] = dim
obs['fct'] = 'ort'
obs.update(info)
res.append(obs)
if tf_add is not None:
# tensorflow
ctx['fct'] = lambda x, y: tf_add(tf_add(tf_add(x, y), y), y)
ctx['xs'] = [convert_to_tensor(x) for x in xs]
ctx['ys'] = [convert_to_tensor(y) for y in ys]
obs = measure_time(
"loop_fct(fct, xs, ys)",
div_by_number=True, context=ctx, repeat=repeat, number=number)
obs['dim'] = dim
obs['fct'] = 'tf'
obs.update(info)
res.append(obs)
if torch_add is not None:
# torch
ctx['fct'] = lambda x, y: torch_add(
torch_add(torch_add(x, y), y), y)
ctx['xs'] = [from_numpy(x) for x in xs]
ctx['ys'] = [from_numpy(y) for y in ys]
obs = measure_time(
"loop_fct(fct, xs, ys)",
div_by_number=True, context=ctx, repeat=repeat, number=number)
obs['dim'] = dim
obs['fct'] = 'torch'
obs.update(info)
res.append(obs)
# Dataframes
shape1_name = str(shape1).replace(str(dim), "N")
shape2_name = str(shape2).replace(str(dim), "N")
df = pandas.DataFrame(res)
df.columns = [_.replace('dim', 'N') for _ in df.columns]
piv = df.pivot('N', 'fct', 'average')
rs = piv.copy()
for c in ['ort', 'torch', 'tf']:
if c in rs.columns:
rs[c] = rs['numpy'] / rs[c]
rs['numpy'] = 1.
# Graphs.
fig, ax = plt.subplots(1, 2, figsize=(12, 4))
piv.plot(logx=True, logy=True, ax=ax[0],
title="%s benchmark\n%s + %s"
" lower better" % (name, shape1_name, shape2_name))
ax[0].legend(prop={"size": 9})
rs.plot(logx=True, logy=True, ax=ax[1],
title="%s Speedup, baseline=numpy\n%s + %s"
" higher better" % (name, shape1_name, shape2_name))
ax[1].plot([min(rs.index), max(rs.index)], [0.5, 0.5], 'g--')
ax[1].plot([min(rs.index), max(rs.index)], [2., 2.], 'g--')
ax[1].legend(prop={"size": 9})
return df, rs, ax
dfs = []
(5, N, N) + (5, N, N)#
df, piv, ax = benchmark_op()
dfs.append(df)
df.pivot("fct", "N", "average")
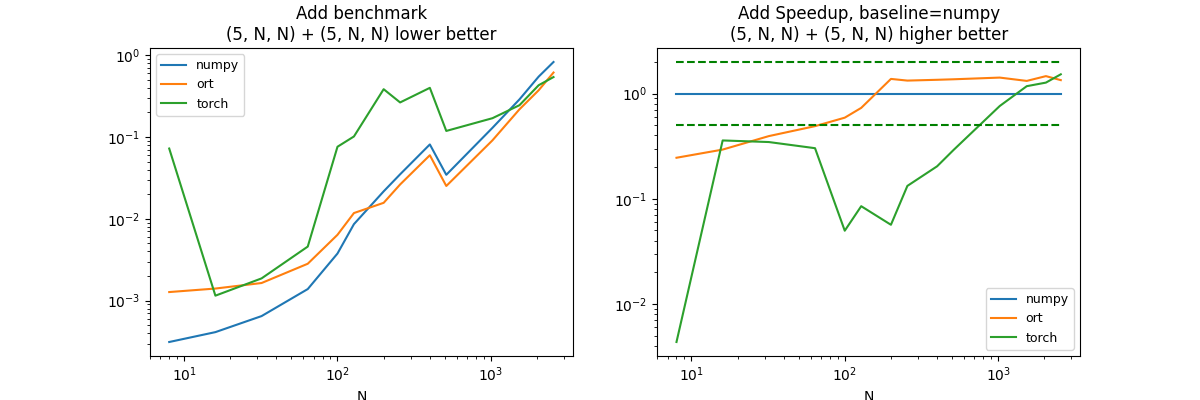
Out:
0%| | 0/14 [00:00<?, ?it/s]
7%|7 | 1/14 [00:00<00:09, 1.31it/s]
29%|##8 | 4/14 [00:00<00:01, 5.04it/s]
36%|###5 | 5/14 [00:01<00:03, 2.51it/s]
43%|####2 | 6/14 [00:03<00:05, 1.52it/s]
50%|##### | 7/14 [00:07<00:12, 1.75s/it]
57%|#####7 | 8/14 [00:11<00:13, 2.29s/it]
64%|######4 | 9/14 [00:17<00:17, 3.45s/it]
71%|#######1 | 10/14 [00:19<00:12, 3.06s/it]
79%|#######8 | 11/14 [00:24<00:11, 3.71s/it]
86%|########5 | 12/14 [00:35<00:11, 5.76s/it]
93%|#########2| 13/14 [00:56<00:10, 10.37s/it]
100%|##########| 14/14 [02:07<00:00, 28.40s/it]
100%|##########| 14/14 [02:07<00:00, 9.10s/it]
(5, N, N) + (5, N, 1)#
shape_fcts = (lambda dim: (5, dim, dim),
lambda dim: (5, dim, 1))
df, piv, ax = benchmark_op(shape_fcts=shape_fcts)
dfs.append(df)
df.pivot("fct", "N", "average")
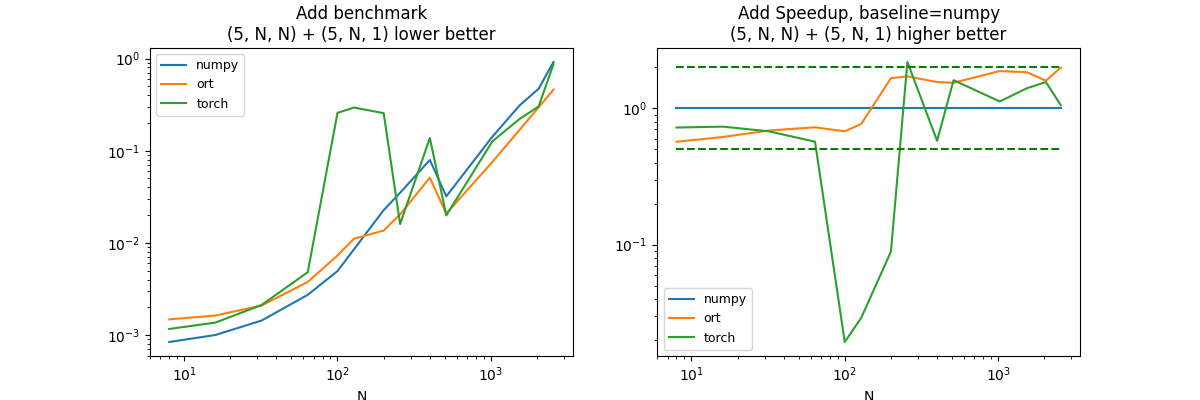
Out:
0%| | 0/14 [00:00<?, ?it/s]
21%|##1 | 3/14 [00:00<00:00, 20.36it/s]
43%|####2 | 6/14 [00:06<00:09, 1.20s/it]
50%|##### | 7/14 [00:09<00:11, 1.60s/it]
57%|#####7 | 8/14 [00:10<00:08, 1.43s/it]
64%|######4 | 9/14 [00:13<00:09, 1.86s/it]
71%|#######1 | 10/14 [00:14<00:06, 1.61s/it]
79%|#######8 | 11/14 [00:18<00:06, 2.30s/it]
86%|########5 | 12/14 [00:26<00:08, 4.06s/it]
93%|#########2| 13/14 [00:40<00:06, 6.76s/it]
100%|##########| 14/14 [01:12<00:00, 14.12s/it]
100%|##########| 14/14 [01:12<00:00, 5.16s/it]
(5, N, N) + (5, 1, N)#
shape_fcts = (lambda dim: (5, dim, dim),
lambda dim: (5, 1, dim))
df, piv, ax = benchmark_op(shape_fcts=shape_fcts)
dfs.append(df)
df.pivot("fct", "N", "average")
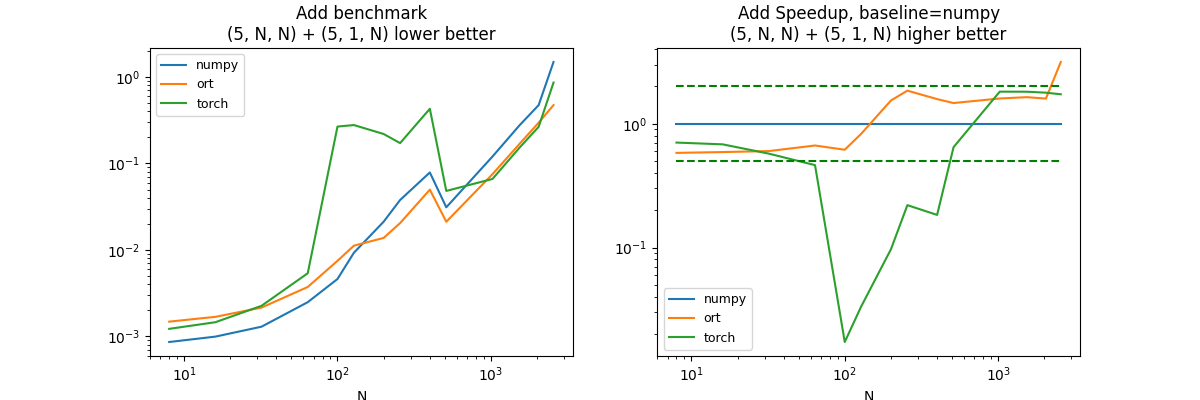
Out:
0%| | 0/14 [00:00<?, ?it/s]
21%|##1 | 3/14 [00:00<00:00, 20.05it/s]
43%|####2 | 6/14 [00:06<00:09, 1.20s/it]
50%|##### | 7/14 [00:08<00:10, 1.52s/it]
57%|#####7 | 8/14 [00:11<00:10, 1.75s/it]
64%|######4 | 9/14 [00:17<00:14, 2.83s/it]
71%|#######1 | 10/14 [00:18<00:09, 2.39s/it]
79%|#######8 | 11/14 [00:21<00:07, 2.64s/it]
86%|########5 | 12/14 [00:29<00:08, 4.02s/it]
93%|#########2| 13/14 [00:43<00:06, 6.94s/it]
100%|##########| 14/14 [01:20<00:00, 15.68s/it]
100%|##########| 14/14 [01:20<00:00, 5.75s/it]
(5, N, 5, N) + (1, N, 1, 1)#
shape_fcts = (lambda dim: (5, dim, 5, dim),
lambda dim: (1, dim, 1, 1))
df, piv, ax = benchmark_op(shape_fcts=shape_fcts)
dfs.append(df)
df.pivot("fct", "N", "average")
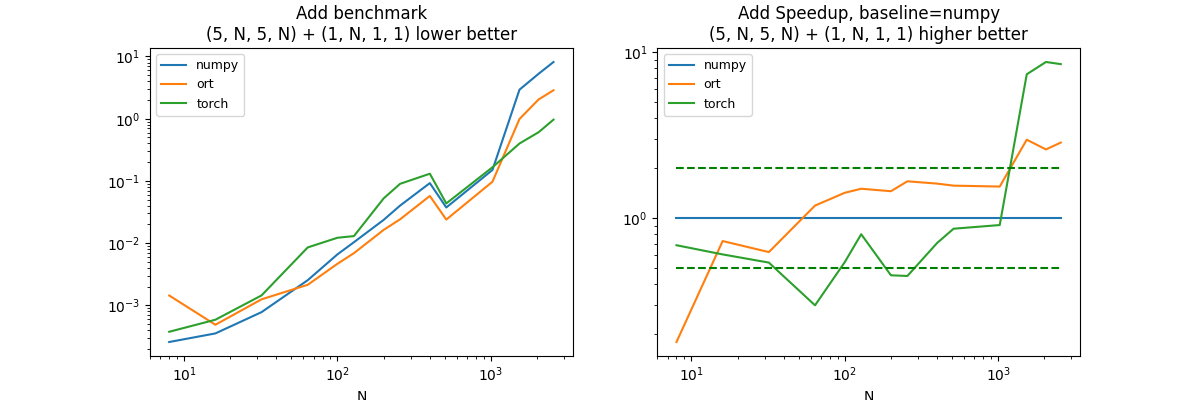
Out:
0%| | 0/14 [00:00<?, ?it/s]
21%|##1 | 3/14 [00:00<00:00, 23.45it/s]
43%|####2 | 6/14 [00:00<00:01, 5.85it/s]
57%|#####7 | 8/14 [00:03<00:03, 1.62it/s]
64%|######4 | 9/14 [00:07<00:05, 1.17s/it]
71%|#######1 | 10/14 [00:08<00:04, 1.19s/it]
79%|#######8 | 11/14 [00:13<00:06, 2.12s/it]
86%|########5 | 12/14 [01:01<00:27, 13.96s/it]
93%|#########2| 13/14 [02:28<00:33, 33.79s/it]
100%|##########| 14/14 [04:45<00:00, 62.29s/it]
100%|##########| 14/14 [04:45<00:00, 20.36s/it]
Conclusion#
It is difficult to have a final conclusion as the addition of two vectors is of the same order of magnitude of a copy between python and the C++ code of onnxruntime, pytorch or tensorflow. numpy is much better of small vectors. onnxruntime, pytorch and tensorflow are not optimized on this case because it is not very common in deep learning.
merged = pandas.concat(dfs)
name = "add"
merged.to_csv("plot_%s.csv" % name, index=False)
merged.to_excel("plot_%s.xlsx" % name, index=False)
plt.savefig("plot_%s.png" % name)
plt.show()
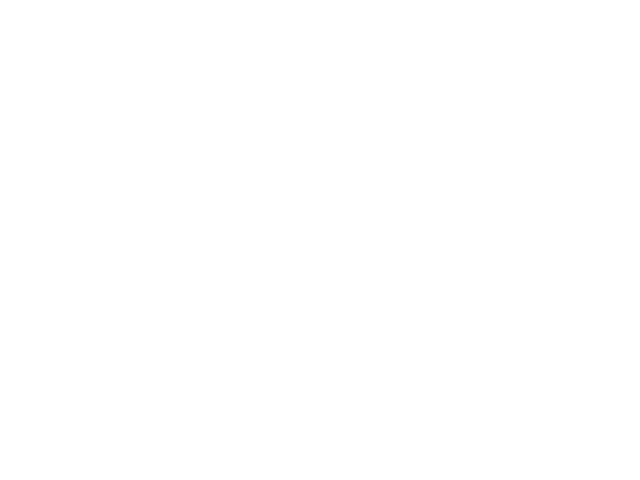
Total running time of the script: ( 9 minutes 45.707 seconds)