Fast TopK elements#
Links: notebook
, html, PDF
, python
, slides, GitHub
Looking for the top k elements is something needed to implement a simple k nearest neighbors. The implementation scikit-learn is using relies on numpy: _kneighbors_reduce_func. mlprodict also contains a C++ implementation of the same function. Let’s compare them.
from jyquickhelper import add_notebook_menu
add_notebook_menu()
%matplotlib inline
Two implementations#
We assume we are looking for the k
nearest elements of every row of
matrix X
which is a dense matrix of doubles.
import numpy.random as rnd
from sklearn.neighbors._base import KNeighborsMixin
mixin = KNeighborsMixin()
def topk_sklearn(X, k):
return mixin._kneighbors_reduce_func(X, 0, k, False)
X = rnd.randn(5, 10)
ind = topk_sklearn(X, 3)
ind
array([[2, 7, 3],
[7, 0, 8],
[1, 5, 6],
[8, 9, 3],
[4, 6, 5]], dtype=int64)
Now the implementation with mlprodict (C++) available at topk_element_min. It uses heap.
from mlprodict.onnxrt.ops_cpu._op_onnx_numpy import topk_element_min_double
def topk_cpp(X, k):
return topk_element_min_double(X, k, True, 50)
ind = topk_cpp(X, 3)
ind
array([[2, 7, 3],
[7, 0, 8],
[1, 5, 6],
[8, 9, 3],
[4, 6, 5]], dtype=int64)
Speed comparison by size#
%timeit topk_sklearn(X, 3)
21.7 µs ± 4.19 µs per loop (mean ± std. dev. of 7 runs, 100000 loops each)
%timeit topk_cpp(X, 3)
4.1 µs ± 435 ns per loop (mean ± std. dev. of 7 runs, 100000 loops each)
Quite a lot faster on this simple example. Let’s look for bigger matrices.
X = rnd.randn(1000, 100)
%timeit topk_sklearn(X, 10)
1.8 ms ± 102 µs per loop (mean ± std. dev. of 7 runs, 1000 loops each)
%timeit topk_cpp(X, 10)
786 µs ± 116 µs per loop (mean ± std. dev. of 7 runs, 1000 loops each)
from cpyquickhelper.numbers import measure_time
from tqdm import tqdm
from pandas import DataFrame
rows = []
for n in tqdm(range(1000, 10001, 1000)):
X = rnd.randn(n, 1000)
res = measure_time('topk_sklearn(X, 20)',
{'X': X, 'topk_sklearn': topk_sklearn},
div_by_number=True,
number=2, repeat=2)
res["N"] = n
res["name"] = 'topk_sklearn'
rows.append(res)
res = measure_time('topk_cpp(X, 20)',
{'X': X, 'topk_cpp': topk_cpp},
div_by_number=True,
number=4, repeat=4)
res["N"] = n
res["name"] = 'topk_cpp'
rows.append(res)
df = DataFrame(rows)
df.head()
100%|██████████| 10/10 [00:08<00:00, 1.16it/s]
average | deviation | min_exec | max_exec | repeat | number | context_size | N | name | |
---|---|---|---|---|---|---|---|---|---|
0 | 0.016310 | 0.000260 | 0.016050 | 0.016571 | 2 | 2 | 240 | 1000 | topk_sklearn |
1 | 0.003872 | 0.000501 | 0.003335 | 0.004631 | 4 | 4 | 240 | 1000 | topk_cpp |
2 | 0.034684 | 0.001629 | 0.033055 | 0.036313 | 2 | 2 | 240 | 2000 | topk_sklearn |
3 | 0.006973 | 0.000558 | 0.006307 | 0.007756 | 4 | 4 | 240 | 2000 | topk_cpp |
4 | 0.051934 | 0.000851 | 0.051084 | 0.052785 | 2 | 2 | 240 | 3000 | topk_sklearn |
import matplotlib.pyplot as plt
fig, ax = plt.subplots(1, 2, figsize=(10, 4))
piv = df.pivot("N", "name", "average")
piv.plot(ax=ax[0], logy=True, logx=True)
ax[0].set_title("top 20")
piv["ratio"] = piv["topk_cpp"] / piv["topk_sklearn"]
piv[["ratio"]].plot(ax=ax[1])
ax[1].set_title("ratio");
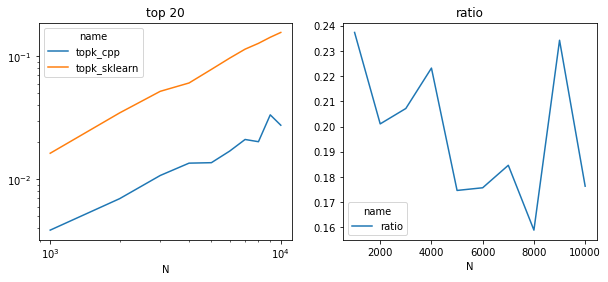
Speed comparison by k#
rows = []
X = rnd.randn(2000, 1000)
for k in tqdm(list(range(1, 20)) + list(range(20, 1000, 20))):
res = measure_time('topk_sklearn(X, k)',
{'X': X, 'topk_sklearn': topk_sklearn, 'k': k},
div_by_number=True,
number=2, repeat=2)
res["k"] = k
res["name"] = 'topk_sklearn'
rows.append(res)
res = measure_time('topk_cpp(X, k)',
{'X': X, 'topk_cpp': topk_cpp, 'k': k},
div_by_number=True,
number=2, repeat=2)
res["k"] = k
res["name"] = 'topk_cpp'
rows.append(res)
df = DataFrame(rows)
df.head()
100%|██████████| 68/68 [00:34<00:00, 1.95it/s]
average | deviation | min_exec | max_exec | repeat | number | context_size | k | name | |
---|---|---|---|---|---|---|---|---|---|
0 | 0.009558 | 0.001392 | 0.008166 | 0.010949 | 2 | 2 | 240 | 1 | topk_sklearn |
1 | 0.002665 | 0.000571 | 0.002094 | 0.003236 | 2 | 2 | 240 | 1 | topk_cpp |
2 | 0.021933 | 0.000575 | 0.021358 | 0.022508 | 2 | 2 | 240 | 2 | topk_sklearn |
3 | 0.001000 | 0.000084 | 0.000917 | 0.001084 | 2 | 2 | 240 | 2 | topk_cpp |
4 | 0.025986 | 0.001411 | 0.024575 | 0.027398 | 2 | 2 | 240 | 3 | topk_sklearn |
fig, ax = plt.subplots(1, 2, figsize=(10, 4))
piv = df.pivot("k", "name", "average")
piv.plot(ax=ax[0], logy=True, logx=True)
ax[0].set_title("top k for 2000 rows")
piv["ratio"] = piv["topk_cpp"] / piv["topk_sklearn"]
piv[["ratio"]].plot(ax=ax[1])
ax[1].set_title("ratio")
ax[0].set_xlabel("k")
ax[1].set_xlabel("k");
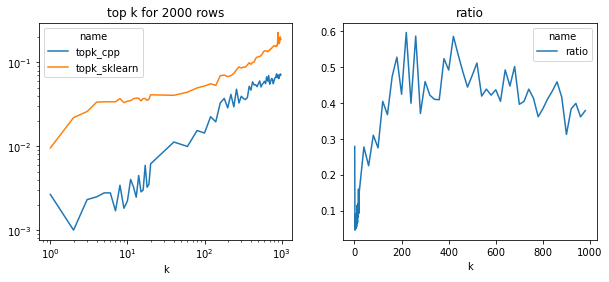
The implementation is half faster in all cases and much more efficient for small values which is usually the case for the nearest neighbors. This implementation is using openmp, maybe that’s why it gets 50% faster on this two cores machine.