Infer operator computation cost#
Links: notebook
, html, PDF
, python
, slides, GitHub
This notebooks explores a way to predict the cost of operator Transpose based on some features.
from jyquickhelper import add_notebook_menu
add_notebook_menu()
%matplotlib inline
%load_ext mlprodict
ONNX graph and measures#
import numpy
from skl2onnx.common.data_types import FloatTensorType
from skl2onnx.algebra.onnx_ops import OnnxTranspose
def create_onnx_graph(perm=(0, 1, 2, 3), target_opset=14):
tr = OnnxTranspose('X', perm=perm, output_names=['Y'], op_version=target_opset)
return tr.to_onnx({'X': FloatTensorType([None] * len(perm))})
onx = create_onnx_graph()
%onnxview onx
from mlprodict.onnxrt import OnnxInference
onx = create_onnx_graph(perm=(1, 0, 3, 2))
oinf = OnnxInference(onx)
inputs = {'X': numpy.full((5, 6, 7, 8), 1, dtype=numpy.float32)}
res = oinf.run(inputs)['Y']
res.shape
(6, 5, 8, 7)
from onnxruntime import InferenceSession
sess = InferenceSession(onx.SerializeToString())
res = sess.run(None, inputs)[0]
res.shape
(6, 5, 8, 7)
from cpyquickhelper.numbers.speed_measure import measure_time
def measure_time_onnx(sess, X, number=50, repeat=30):
inputs = {'X': X}
return measure_time(lambda: sess.run(None, inputs), context=dict(sess=sess, inputs=inputs),
div_by_number=True, number=number, repeat=repeat)
X = numpy.random.random((3, 224, 224, 4)).astype(numpy.float32)
measure_time_onnx(sess, X)
{'average': 0.0024677738666666646,
'deviation': 0.00022911153911864325,
'min_exec': 0.0022292380000000023,
'max_exec': 0.003265080000000005,
'repeat': 30,
'number': 50,
'context_size': 232}
Simulation to build a database#
Many dimensions, many permutations#
from itertools import permutations
from tqdm import tqdm
from pandas import DataFrame
def process_shape(shape, rnd=False, number=50, repeat=30, bar=True):
X = numpy.random.random(shape).astype(numpy.float32)
obs = []
perms = list(permutations(list(range(len(X.shape)))))
baseline = None
itergen = perms if (rnd or not bar) else tqdm(perms)
for perm in itergen:
if baseline is not None and rnd:
if random.randint(0, 4) != 0:
continue
onx = create_onnx_graph(perm=perm)
sess = InferenceSession(onx.SerializeToString())
res = measure_time_onnx(sess, X, number=number, repeat=repeat)
res['perm'] = perm
res['shape'] = shape
if baseline is None:
baseline = res
res["ratio"] = res["average"] / baseline["average"]
res['dim'] = len(shape)
obs.append(res)
return DataFrame(obs).sort_values('average')
dfs = []
df = process_shape((12, 13, 15, 18))
dfs.append(df)
df
100%|██████████| 24/24 [00:04<00:00, 5.73it/s]
average | deviation | min_exec | max_exec | repeat | number | context_size | perm | shape | ratio | dim | |
---|---|---|---|---|---|---|---|---|---|---|---|
3 | 0.000044 | 0.000006 | 0.000039 | 0.000057 | 30 | 50 | 232 | (0, 2, 3, 1) | (12, 13, 15, 18) | 0.750316 | 4 |
1 | 0.000048 | 0.000003 | 0.000045 | 0.000058 | 30 | 50 | 232 | (0, 1, 3, 2) | (12, 13, 15, 18) | 0.820821 | 4 |
18 | 0.000049 | 0.000003 | 0.000045 | 0.000062 | 30 | 50 | 232 | (3, 0, 1, 2) | (12, 13, 15, 18) | 0.823070 | 4 |
9 | 0.000049 | 0.000001 | 0.000048 | 0.000053 | 30 | 50 | 232 | (1, 2, 3, 0) | (12, 13, 15, 18) | 0.830604 | 4 |
12 | 0.000051 | 0.000004 | 0.000039 | 0.000062 | 30 | 50 | 232 | (2, 0, 1, 3) | (12, 13, 15, 18) | 0.861994 | 4 |
4 | 0.000052 | 0.000005 | 0.000047 | 0.000073 | 30 | 50 | 232 | (0, 3, 1, 2) | (12, 13, 15, 18) | 0.889753 | 4 |
8 | 0.000054 | 0.000006 | 0.000044 | 0.000067 | 30 | 50 | 232 | (1, 2, 0, 3) | (12, 13, 15, 18) | 0.909477 | 4 |
2 | 0.000054 | 0.000007 | 0.000049 | 0.000081 | 30 | 50 | 232 | (0, 2, 1, 3) | (12, 13, 15, 18) | 0.922354 | 4 |
14 | 0.000057 | 0.000006 | 0.000046 | 0.000064 | 30 | 50 | 232 | (2, 1, 0, 3) | (12, 13, 15, 18) | 0.972198 | 4 |
0 | 0.000059 | 0.000019 | 0.000034 | 0.000093 | 30 | 50 | 232 | (0, 1, 2, 3) | (12, 13, 15, 18) | 1.000000 | 4 |
6 | 0.000092 | 0.000019 | 0.000053 | 0.000139 | 30 | 50 | 232 | (1, 0, 2, 3) | (12, 13, 15, 18) | 1.557903 | 4 |
11 | 0.000136 | 0.000020 | 0.000119 | 0.000186 | 30 | 50 | 232 | (1, 3, 2, 0) | (12, 13, 15, 18) | 2.301556 | 4 |
13 | 0.000138 | 0.000023 | 0.000121 | 0.000181 | 30 | 50 | 232 | (2, 0, 3, 1) | (12, 13, 15, 18) | 2.336826 | 4 |
10 | 0.000138 | 0.000018 | 0.000118 | 0.000176 | 30 | 50 | 232 | (1, 3, 0, 2) | (12, 13, 15, 18) | 2.346118 | 4 |
16 | 0.000140 | 0.000015 | 0.000124 | 0.000193 | 30 | 50 | 232 | (2, 3, 0, 1) | (12, 13, 15, 18) | 2.379168 | 4 |
15 | 0.000144 | 0.000019 | 0.000119 | 0.000196 | 30 | 50 | 232 | (2, 1, 3, 0) | (12, 13, 15, 18) | 2.443392 | 4 |
17 | 0.000145 | 0.000022 | 0.000123 | 0.000199 | 30 | 50 | 232 | (2, 3, 1, 0) | (12, 13, 15, 18) | 2.455098 | 4 |
23 | 0.000145 | 0.000017 | 0.000125 | 0.000196 | 30 | 50 | 232 | (3, 2, 1, 0) | (12, 13, 15, 18) | 2.456431 | 4 |
20 | 0.000146 | 0.000015 | 0.000128 | 0.000184 | 30 | 50 | 232 | (3, 1, 0, 2) | (12, 13, 15, 18) | 2.473250 | 4 |
22 | 0.000150 | 0.000017 | 0.000127 | 0.000170 | 30 | 50 | 232 | (3, 2, 0, 1) | (12, 13, 15, 18) | 2.539817 | 4 |
19 | 0.000158 | 0.000021 | 0.000127 | 0.000192 | 30 | 50 | 232 | (3, 0, 2, 1) | (12, 13, 15, 18) | 2.684876 | 4 |
21 | 0.000164 | 0.000045 | 0.000124 | 0.000231 | 30 | 50 | 232 | (3, 1, 2, 0) | (12, 13, 15, 18) | 2.778193 | 4 |
7 | 0.000214 | 0.000060 | 0.000136 | 0.000295 | 30 | 50 | 232 | (1, 0, 3, 2) | (12, 13, 15, 18) | 3.627240 | 4 |
5 | 0.000215 | 0.000071 | 0.000143 | 0.000340 | 30 | 50 | 232 | (0, 3, 2, 1) | (12, 13, 15, 18) | 3.640132 | 4 |
df = process_shape((43, 44, 45))
dfs.append(df)
df
100%|██████████| 6/6 [00:01<00:00, 4.70it/s]
average | deviation | min_exec | max_exec | repeat | number | context_size | perm | shape | ratio | dim | |
---|---|---|---|---|---|---|---|---|---|---|---|
3 | 0.000073 | 0.000009 | 0.000062 | 0.000094 | 30 | 50 | 232 | (1, 2, 0) | (43, 44, 45) | 0.985513 | 3 |
0 | 0.000074 | 0.000009 | 0.000065 | 0.000109 | 30 | 50 | 232 | (0, 1, 2) | (43, 44, 45) | 1.000000 | 3 |
1 | 0.000077 | 0.000008 | 0.000069 | 0.000101 | 30 | 50 | 232 | (0, 2, 1) | (43, 44, 45) | 1.032759 | 3 |
4 | 0.000097 | 0.000004 | 0.000083 | 0.000110 | 30 | 50 | 232 | (2, 0, 1) | (43, 44, 45) | 1.300915 | 3 |
2 | 0.000113 | 0.000029 | 0.000061 | 0.000141 | 30 | 50 | 232 | (1, 0, 2) | (43, 44, 45) | 1.515711 | 3 |
5 | 0.000375 | 0.000121 | 0.000292 | 0.000750 | 30 | 50 | 232 | (2, 1, 0) | (43, 44, 45) | 5.054301 | 3 |
df = process_shape((3, 244, 244))
dfs.append(df)
df
100%|██████████| 6/6 [00:01<00:00, 3.05it/s]
average | deviation | min_exec | max_exec | repeat | number | context_size | perm | shape | ratio | dim | |
---|---|---|---|---|---|---|---|---|---|---|---|
2 | 0.000100 | 0.000009 | 0.000090 | 0.000125 | 30 | 50 | 232 | (1, 0, 2) | (3, 244, 244) | 0.955203 | 3 |
0 | 0.000105 | 0.000016 | 0.000078 | 0.000138 | 30 | 50 | 232 | (0, 1, 2) | (3, 244, 244) | 1.000000 | 3 |
1 | 0.000123 | 0.000013 | 0.000108 | 0.000161 | 30 | 50 | 232 | (0, 2, 1) | (3, 244, 244) | 1.178827 | 3 |
4 | 0.000124 | 0.000017 | 0.000108 | 0.000171 | 30 | 50 | 232 | (2, 0, 1) | (3, 244, 244) | 1.185666 | 3 |
3 | 0.000151 | 0.000016 | 0.000136 | 0.000197 | 30 | 50 | 232 | (1, 2, 0) | (3, 244, 244) | 1.438446 | 3 |
5 | 0.000672 | 0.000083 | 0.000626 | 0.001030 | 30 | 50 | 232 | (2, 1, 0) | (3, 244, 244) | 6.418195 | 3 |
df = process_shape((3, 244, 244, 1))
dfs.append(df)
df
100%|██████████| 24/24 [00:19<00:00, 1.26it/s]
average | deviation | min_exec | max_exec | repeat | number | context_size | perm | shape | ratio | dim | |
---|---|---|---|---|---|---|---|---|---|---|---|
4 | 0.000092 | 0.000008 | 0.000078 | 0.000107 | 30 | 50 | 232 | (0, 3, 1, 2) | (3, 244, 244, 1) | 0.859903 | 4 |
0 | 0.000107 | 0.000018 | 0.000084 | 0.000157 | 30 | 50 | 232 | (0, 1, 2, 3) | (3, 244, 244, 1) | 1.000000 | 4 |
6 | 0.000124 | 0.000068 | 0.000088 | 0.000323 | 30 | 50 | 232 | (1, 0, 2, 3) | (3, 244, 244, 1) | 1.162456 | 4 |
12 | 0.000126 | 0.000017 | 0.000107 | 0.000185 | 30 | 50 | 232 | (2, 0, 1, 3) | (3, 244, 244, 1) | 1.180996 | 4 |
3 | 0.000130 | 0.000009 | 0.000120 | 0.000163 | 30 | 50 | 232 | (0, 2, 3, 1) | (3, 244, 244, 1) | 1.210077 | 4 |
18 | 0.000137 | 0.000047 | 0.000090 | 0.000250 | 30 | 50 | 232 | (3, 0, 1, 2) | (3, 244, 244, 1) | 1.276642 | 4 |
1 | 0.000147 | 0.000017 | 0.000106 | 0.000175 | 30 | 50 | 232 | (0, 1, 3, 2) | (3, 244, 244, 1) | 1.369978 | 4 |
8 | 0.000185 | 0.000017 | 0.000164 | 0.000246 | 30 | 50 | 232 | (1, 2, 0, 3) | (3, 244, 244, 1) | 1.725391 | 4 |
9 | 0.000189 | 0.000044 | 0.000142 | 0.000265 | 30 | 50 | 232 | (1, 2, 3, 0) | (3, 244, 244, 1) | 1.766905 | 4 |
2 | 0.000201 | 0.000054 | 0.000121 | 0.000289 | 30 | 50 | 232 | (0, 2, 1, 3) | (3, 244, 244, 1) | 1.878802 | 4 |
7 | 0.000522 | 0.000061 | 0.000457 | 0.000733 | 30 | 50 | 232 | (1, 0, 3, 2) | (3, 244, 244, 1) | 4.874009 | 4 |
10 | 0.000533 | 0.000157 | 0.000456 | 0.001128 | 30 | 50 | 232 | (1, 3, 0, 2) | (3, 244, 244, 1) | 4.973916 | 4 |
13 | 0.000640 | 0.000189 | 0.000477 | 0.001289 | 30 | 50 | 232 | (2, 0, 3, 1) | (3, 244, 244, 1) | 5.980796 | 4 |
16 | 0.000660 | 0.000106 | 0.000503 | 0.000860 | 30 | 50 | 232 | (2, 3, 0, 1) | (3, 244, 244, 1) | 6.167703 | 4 |
5 | 0.000692 | 0.000136 | 0.000529 | 0.001021 | 30 | 50 | 232 | (0, 3, 2, 1) | (3, 244, 244, 1) | 6.460759 | 4 |
19 | 0.000749 | 0.000206 | 0.000508 | 0.001324 | 30 | 50 | 232 | (3, 0, 2, 1) | (3, 244, 244, 1) | 6.996362 | 4 |
14 | 0.000754 | 0.000105 | 0.000633 | 0.000994 | 30 | 50 | 232 | (2, 1, 0, 3) | (3, 244, 244, 1) | 7.041007 | 4 |
11 | 0.000791 | 0.000264 | 0.000561 | 0.001386 | 30 | 50 | 232 | (1, 3, 2, 0) | (3, 244, 244, 1) | 7.389431 | 4 |
15 | 0.000818 | 0.000278 | 0.000625 | 0.001522 | 30 | 50 | 232 | (2, 1, 3, 0) | (3, 244, 244, 1) | 7.634646 | 4 |
17 | 0.000893 | 0.000212 | 0.000646 | 0.001477 | 30 | 50 | 232 | (2, 3, 1, 0) | (3, 244, 244, 1) | 8.339926 | 4 |
21 | 0.000944 | 0.000293 | 0.000581 | 0.001626 | 30 | 50 | 232 | (3, 1, 2, 0) | (3, 244, 244, 1) | 8.814785 | 4 |
20 | 0.000976 | 0.000347 | 0.000584 | 0.001742 | 30 | 50 | 232 | (3, 1, 0, 2) | (3, 244, 244, 1) | 9.112243 | 4 |
22 | 0.001011 | 0.000337 | 0.000544 | 0.001810 | 30 | 50 | 232 | (3, 2, 0, 1) | (3, 244, 244, 1) | 9.437403 | 4 |
23 | 0.001128 | 0.000322 | 0.000629 | 0.001737 | 30 | 50 | 232 | (3, 2, 1, 0) | (3, 244, 244, 1) | 10.530182 | 4 |
df = process_shape((1, 244, 244, 3))
dfs.append(df)
df
100%|██████████| 24/24 [00:22<00:00, 1.07it/s]
average | deviation | min_exec | max_exec | repeat | number | context_size | perm | shape | ratio | dim | |
---|---|---|---|---|---|---|---|---|---|---|---|
8 | 0.000092 | 0.000014 | 0.000078 | 0.000132 | 30 | 50 | 232 | (1, 2, 0, 3) | (1, 244, 244, 3) | 0.753009 | 4 |
6 | 0.000098 | 0.000013 | 0.000083 | 0.000142 | 30 | 50 | 232 | (1, 0, 2, 3) | (1, 244, 244, 3) | 0.802808 | 4 |
9 | 0.000107 | 0.000018 | 0.000075 | 0.000137 | 30 | 50 | 232 | (1, 2, 3, 0) | (1, 244, 244, 3) | 0.873932 | 4 |
3 | 0.000115 | 0.000015 | 0.000092 | 0.000147 | 30 | 50 | 232 | (0, 2, 3, 1) | (1, 244, 244, 3) | 0.940606 | 4 |
0 | 0.000122 | 0.000028 | 0.000094 | 0.000201 | 30 | 50 | 232 | (0, 1, 2, 3) | (1, 244, 244, 3) | 1.000000 | 4 |
1 | 0.000194 | 0.000036 | 0.000160 | 0.000311 | 30 | 50 | 232 | (0, 1, 3, 2) | (1, 244, 244, 3) | 1.585479 | 4 |
4 | 0.000195 | 0.000019 | 0.000163 | 0.000258 | 30 | 50 | 232 | (0, 3, 1, 2) | (1, 244, 244, 3) | 1.598770 | 4 |
18 | 0.000235 | 0.000058 | 0.000172 | 0.000345 | 30 | 50 | 232 | (3, 0, 1, 2) | (1, 244, 244, 3) | 1.923654 | 4 |
2 | 0.000408 | 0.000156 | 0.000229 | 0.000718 | 30 | 50 | 232 | (0, 2, 1, 3) | (1, 244, 244, 3) | 3.345406 | 4 |
12 | 0.000513 | 0.000215 | 0.000300 | 0.001430 | 30 | 50 | 232 | (2, 0, 1, 3) | (1, 244, 244, 3) | 4.205477 | 4 |
10 | 0.000558 | 0.000131 | 0.000458 | 0.001023 | 30 | 50 | 232 | (1, 3, 0, 2) | (1, 244, 244, 3) | 4.572658 | 4 |
7 | 0.000604 | 0.000188 | 0.000471 | 0.001065 | 30 | 50 | 232 | (1, 0, 3, 2) | (1, 244, 244, 3) | 4.947937 | 4 |
14 | 0.000620 | 0.000142 | 0.000410 | 0.001121 | 30 | 50 | 232 | (2, 1, 0, 3) | (1, 244, 244, 3) | 5.078387 | 4 |
23 | 0.000679 | 0.000097 | 0.000590 | 0.000928 | 30 | 50 | 232 | (3, 2, 1, 0) | (1, 244, 244, 3) | 5.561888 | 4 |
22 | 0.000710 | 0.000161 | 0.000620 | 0.001390 | 30 | 50 | 232 | (3, 2, 0, 1) | (1, 244, 244, 3) | 5.818089 | 4 |
17 | 0.000737 | 0.000240 | 0.000493 | 0.001174 | 30 | 50 | 232 | (2, 3, 1, 0) | (1, 244, 244, 3) | 6.040189 | 4 |
11 | 0.000824 | 0.000288 | 0.000515 | 0.001879 | 30 | 50 | 232 | (1, 3, 2, 0) | (1, 244, 244, 3) | 6.752663 | 4 |
21 | 0.000913 | 0.000216 | 0.000613 | 0.001410 | 30 | 50 | 232 | (3, 1, 2, 0) | (1, 244, 244, 3) | 7.476378 | 4 |
20 | 0.000918 | 0.000328 | 0.000572 | 0.002079 | 30 | 50 | 232 | (3, 1, 0, 2) | (1, 244, 244, 3) | 7.521481 | 4 |
16 | 0.001057 | 0.000609 | 0.000502 | 0.002702 | 30 | 50 | 232 | (2, 3, 0, 1) | (1, 244, 244, 3) | 8.657076 | 4 |
5 | 0.001061 | 0.000612 | 0.000539 | 0.003790 | 30 | 50 | 232 | (0, 3, 2, 1) | (1, 244, 244, 3) | 8.693870 | 4 |
19 | 0.001212 | 0.000417 | 0.000719 | 0.002561 | 30 | 50 | 232 | (3, 0, 2, 1) | (1, 244, 244, 3) | 9.929308 | 4 |
15 | 0.001311 | 0.000505 | 0.000856 | 0.003377 | 30 | 50 | 232 | (2, 1, 3, 0) | (1, 244, 244, 3) | 10.739398 | 4 |
13 | 0.001433 | 0.000505 | 0.000721 | 0.002335 | 30 | 50 | 232 | (2, 0, 3, 1) | (1, 244, 244, 3) | 11.740772 | 4 |
df = process_shape((3, 244, 244, 3), number=15, repeat=15)
dfs.append(df)
df
100%|██████████| 24/24 [00:14<00:00, 1.62it/s]
average | deviation | min_exec | max_exec | repeat | number | context_size | perm | shape | ratio | dim | |
---|---|---|---|---|---|---|---|---|---|---|---|
0 | 0.001088 | 0.000085 | 0.000986 | 0.001291 | 15 | 15 | 232 | (0, 1, 2, 3) | (3, 244, 244, 3) | 1.000000 | 4 |
4 | 0.001227 | 0.000088 | 0.001152 | 0.001474 | 15 | 15 | 232 | (0, 3, 1, 2) | (3, 244, 244, 3) | 1.128126 | 4 |
18 | 0.001277 | 0.000118 | 0.001079 | 0.001490 | 15 | 15 | 232 | (3, 0, 1, 2) | (3, 244, 244, 3) | 1.173721 | 4 |
6 | 0.001311 | 0.000320 | 0.001007 | 0.001925 | 15 | 15 | 232 | (1, 0, 2, 3) | (3, 244, 244, 3) | 1.205182 | 4 |
1 | 0.001415 | 0.000307 | 0.001200 | 0.002498 | 15 | 15 | 232 | (0, 1, 3, 2) | (3, 244, 244, 3) | 1.300901 | 4 |
3 | 0.001426 | 0.000221 | 0.001191 | 0.001863 | 15 | 15 | 232 | (0, 2, 3, 1) | (3, 244, 244, 3) | 1.311361 | 4 |
9 | 0.001510 | 0.000432 | 0.001132 | 0.002417 | 15 | 15 | 232 | (1, 2, 3, 0) | (3, 244, 244, 3) | 1.388068 | 4 |
8 | 0.001552 | 0.000030 | 0.001500 | 0.001602 | 15 | 15 | 232 | (1, 2, 0, 3) | (3, 244, 244, 3) | 1.427105 | 4 |
12 | 0.001724 | 0.000193 | 0.001470 | 0.002142 | 15 | 15 | 232 | (2, 0, 1, 3) | (3, 244, 244, 3) | 1.585155 | 4 |
2 | 0.001790 | 0.000191 | 0.001566 | 0.002238 | 15 | 15 | 232 | (0, 2, 1, 3) | (3, 244, 244, 3) | 1.645717 | 4 |
7 | 0.002528 | 0.000154 | 0.002327 | 0.002983 | 15 | 15 | 232 | (1, 0, 3, 2) | (3, 244, 244, 3) | 2.324384 | 4 |
19 | 0.002571 | 0.000186 | 0.002383 | 0.002922 | 15 | 15 | 232 | (3, 0, 2, 1) | (3, 244, 244, 3) | 2.363443 | 4 |
21 | 0.002591 | 0.000253 | 0.002431 | 0.003403 | 15 | 15 | 232 | (3, 1, 2, 0) | (3, 244, 244, 3) | 2.381860 | 4 |
22 | 0.002698 | 0.000412 | 0.002346 | 0.003689 | 15 | 15 | 232 | (3, 2, 0, 1) | (3, 244, 244, 3) | 2.480308 | 4 |
20 | 0.002806 | 0.000783 | 0.002147 | 0.004296 | 15 | 15 | 232 | (3, 1, 0, 2) | (3, 244, 244, 3) | 2.579517 | 4 |
16 | 0.003212 | 0.000304 | 0.002773 | 0.003851 | 15 | 15 | 232 | (2, 3, 0, 1) | (3, 244, 244, 3) | 2.953032 | 4 |
14 | 0.003228 | 0.000796 | 0.002071 | 0.004791 | 15 | 15 | 232 | (2, 1, 0, 3) | (3, 244, 244, 3) | 2.967523 | 4 |
11 | 0.003257 | 0.000287 | 0.002912 | 0.003739 | 15 | 15 | 232 | (1, 3, 2, 0) | (3, 244, 244, 3) | 2.994043 | 4 |
17 | 0.003574 | 0.000479 | 0.003028 | 0.005042 | 15 | 15 | 232 | (2, 3, 1, 0) | (3, 244, 244, 3) | 3.285842 | 4 |
10 | 0.003942 | 0.001860 | 0.002446 | 0.008241 | 15 | 15 | 232 | (1, 3, 0, 2) | (3, 244, 244, 3) | 3.624145 | 4 |
15 | 0.004249 | 0.001217 | 0.003175 | 0.008041 | 15 | 15 | 232 | (2, 1, 3, 0) | (3, 244, 244, 3) | 3.906361 | 4 |
5 | 0.004685 | 0.001343 | 0.002827 | 0.006868 | 15 | 15 | 232 | (0, 3, 2, 1) | (3, 244, 244, 3) | 4.307072 | 4 |
13 | 0.005539 | 0.002180 | 0.002991 | 0.009602 | 15 | 15 | 232 | (2, 0, 3, 1) | (3, 244, 244, 3) | 5.092422 | 4 |
23 | 0.005575 | 0.001930 | 0.002876 | 0.008157 | 15 | 15 | 232 | (3, 2, 1, 0) | (3, 244, 244, 3) | 5.125597 | 4 |
df = process_shape((3, 244, 244, 6), number=15, repeat=15)
dfs.append(df)
df
100%|██████████| 24/24 [00:34<00:00, 1.43s/it]
average | deviation | min_exec | max_exec | repeat | number | context_size | perm | shape | ratio | dim | |
---|---|---|---|---|---|---|---|---|---|---|---|
1 | 0.002249 | 0.000144 | 0.002067 | 0.002627 | 15 | 15 | 232 | (0, 1, 3, 2) | (3, 244, 244, 6) | 0.606961 | 4 |
3 | 0.002711 | 0.000171 | 0.002458 | 0.002995 | 15 | 15 | 232 | (0, 2, 3, 1) | (3, 244, 244, 6) | 0.731795 | 4 |
12 | 0.002773 | 0.000683 | 0.002260 | 0.004103 | 15 | 15 | 232 | (2, 0, 1, 3) | (3, 244, 244, 6) | 0.748578 | 4 |
4 | 0.002953 | 0.000677 | 0.002187 | 0.004132 | 15 | 15 | 232 | (0, 3, 1, 2) | (3, 244, 244, 6) | 0.797062 | 4 |
2 | 0.003232 | 0.000963 | 0.002303 | 0.005088 | 15 | 15 | 232 | (0, 2, 1, 3) | (3, 244, 244, 6) | 0.872427 | 4 |
6 | 0.003363 | 0.000372 | 0.002883 | 0.004025 | 15 | 15 | 232 | (1, 0, 2, 3) | (3, 244, 244, 6) | 0.907834 | 4 |
8 | 0.003397 | 0.000237 | 0.002886 | 0.003846 | 15 | 15 | 232 | (1, 2, 0, 3) | (3, 244, 244, 6) | 0.917011 | 4 |
9 | 0.003653 | 0.000874 | 0.002567 | 0.005244 | 15 | 15 | 232 | (1, 2, 3, 0) | (3, 244, 244, 6) | 0.986071 | 4 |
14 | 0.003697 | 0.000186 | 0.003495 | 0.004150 | 15 | 15 | 232 | (2, 1, 0, 3) | (3, 244, 244, 6) | 0.997901 | 4 |
0 | 0.003705 | 0.000797 | 0.002111 | 0.005164 | 15 | 15 | 232 | (0, 1, 2, 3) | (3, 244, 244, 6) | 1.000000 | 4 |
18 | 0.003780 | 0.000882 | 0.002701 | 0.005402 | 15 | 15 | 232 | (3, 0, 1, 2) | (3, 244, 244, 6) | 1.020432 | 4 |
10 | 0.004938 | 0.000367 | 0.004532 | 0.005844 | 15 | 15 | 232 | (1, 3, 0, 2) | (3, 244, 244, 6) | 1.333061 | 4 |
7 | 0.005918 | 0.001085 | 0.004598 | 0.008312 | 15 | 15 | 232 | (1, 0, 3, 2) | (3, 244, 244, 6) | 1.597357 | 4 |
13 | 0.006106 | 0.000556 | 0.005619 | 0.007305 | 15 | 15 | 232 | (2, 0, 3, 1) | (3, 244, 244, 6) | 1.648325 | 4 |
11 | 0.006722 | 0.001807 | 0.005067 | 0.011245 | 15 | 15 | 232 | (1, 3, 2, 0) | (3, 244, 244, 6) | 1.814552 | 4 |
20 | 0.007071 | 0.000982 | 0.005454 | 0.008559 | 15 | 15 | 232 | (3, 1, 0, 2) | (3, 244, 244, 6) | 1.908667 | 4 |
21 | 0.007441 | 0.001732 | 0.006199 | 0.012169 | 15 | 15 | 232 | (3, 1, 2, 0) | (3, 244, 244, 6) | 2.008635 | 4 |
15 | 0.007815 | 0.001757 | 0.005932 | 0.010779 | 15 | 15 | 232 | (2, 1, 3, 0) | (3, 244, 244, 6) | 2.109489 | 4 |
16 | 0.008546 | 0.001384 | 0.005878 | 0.010614 | 15 | 15 | 232 | (2, 3, 0, 1) | (3, 244, 244, 6) | 2.306951 | 4 |
5 | 0.010339 | 0.002789 | 0.005878 | 0.018301 | 15 | 15 | 232 | (0, 3, 2, 1) | (3, 244, 244, 6) | 2.790823 | 4 |
17 | 0.010677 | 0.001457 | 0.008504 | 0.014070 | 15 | 15 | 232 | (2, 3, 1, 0) | (3, 244, 244, 6) | 2.882191 | 4 |
23 | 0.012421 | 0.003052 | 0.007818 | 0.018106 | 15 | 15 | 232 | (3, 2, 1, 0) | (3, 244, 244, 6) | 3.352770 | 4 |
22 | 0.013432 | 0.004496 | 0.006536 | 0.021250 | 15 | 15 | 232 | (3, 2, 0, 1) | (3, 244, 244, 6) | 3.625680 | 4 |
19 | 0.014579 | 0.004026 | 0.007144 | 0.020739 | 15 | 15 | 232 | (3, 0, 2, 1) | (3, 244, 244, 6) | 3.935483 | 4 |
Random cases#
import random
if False: # comment out for more training data
for i in tqdm(range(0, 30)):
dim = random.randint(3, 5)
total = 1e8
while total > 1e6 or total < 0:
if dim == 3:
shape = [random.randint(3, 64), random.randint(3, 224), random.randint(3, 64)]
elif dim == 4:
shape = (
[random.randint(3, 8)] +
[random.randint(16, 224) for d in range(2)] +
[random.randint(16, 64)])
elif dim == 5:
shape = (
[random.randint(3, 8)] +
[random.randint(16, 32) for d in range(3)] +
[random.randint(16, 64)])
else:
raise NotImplementedError()
ashape = numpy.array(shape, dtype=numpy.float64)
total = numpy.prod(ashape)
if total > 1000000:
number, repeat = 2, 2
elif total > 800000:
number, repeat = 3, 3
elif total > 500000:
number, repeat = 5, 5
elif total > 200000:
number, repeat = 7, 7
else:
number, repeat = 10, 10
df = process_shape(tuple(shape), number=number, repeat=repeat, bar=False)
dfs.append(df)
for i in range(len(shape)):
shape2 = shape.copy()
shape2[i] = 1
df = process_shape(tuple(shape), number=number, repeat=repeat, bar=False)
dfs.append(df)
len(dfs)
7
import pandas
data = pandas.concat(dfs, axis=0).reset_index(drop=True)
data.tail()
average | deviation | min_exec | max_exec | repeat | number | context_size | perm | shape | ratio | dim | |
---|---|---|---|---|---|---|---|---|---|---|---|
127 | 0.010339 | 0.002789 | 0.005878 | 0.018301 | 15 | 15 | 232 | (0, 3, 2, 1) | (3, 244, 244, 6) | 2.790823 | 4 |
128 | 0.010677 | 0.001457 | 0.008504 | 0.014070 | 15 | 15 | 232 | (2, 3, 1, 0) | (3, 244, 244, 6) | 2.882191 | 4 |
129 | 0.012421 | 0.003052 | 0.007818 | 0.018106 | 15 | 15 | 232 | (3, 2, 1, 0) | (3, 244, 244, 6) | 3.352770 | 4 |
130 | 0.013432 | 0.004496 | 0.006536 | 0.021250 | 15 | 15 | 232 | (3, 2, 0, 1) | (3, 244, 244, 6) | 3.625680 | 4 |
131 | 0.014579 | 0.004026 | 0.007144 | 0.020739 | 15 | 15 | 232 | (3, 0, 2, 1) | (3, 244, 244, 6) | 3.935483 | 4 |
data.shape
(132, 11)
data[['dim', 'shape', 'ratio']].groupby(['dim', 'shape']).agg({'ratio': [min, max, numpy.mean, numpy.median]})
ratio | |||||
---|---|---|---|---|---|
min | max | mean | median | ||
dim | shape | ||||
3 | (3, 244, 244) | 0.955203 | 6.418195 | 2.029389 | 1.182247 |
(43, 44, 45) | 0.985513 | 5.054301 | 1.814867 | 1.166837 | |
4 | (1, 244, 244, 3) | 0.753009 | 11.740772 | 5.023301 | 5.013162 |
(3, 244, 244, 1) | 0.859903 | 10.530182 | 4.882680 | 5.477356 | |
(3, 244, 244, 3) | 1.000000 | 5.125597 | 2.481287 | 2.372651 | |
(3, 244, 244, 6) | 0.606961 | 3.935483 | 1.704169 | 1.465209 | |
(12, 13, 15, 18) | 0.750316 | 3.640132 | 1.866691 | 2.319191 |
features#
Computing the features#
def _edit_distance(mot1, mot2):
dist = {(-1, -1): 0}
pred = {(-1, -1): None}
if len(mot1) == 0:
for j, d in enumerate(mot2):
dist[-1, j] = dist[-1, j - 1] + 1
pred[-1, j] = (-1, j - 1)
dist[j, -1] = dist[j - 1, -1] + 1
pred[j, -1] = (j - 1, -1)
for i, c in enumerate(mot1):
dist[i, -1] = dist[i - 1, -1] + 1
pred[i, -1] = (i - 1, -1)
dist[-1, i] = dist[-1, i - 1] + 1
pred[-1, i] = (-1, i - 1)
for j, d in enumerate(mot2):
opt = []
if (i - 1, j) in dist:
x = dist[i - 1, j] + 1
opt.append((x, (i - 1, j)))
if (i, j - 1) in dist:
x = dist[i, j - 1] + 1
opt.append((x, (i, j - 1)))
if (i - 1, j - 1) in dist:
x = dist[i - 1, j - 1] + (1 if c != d else 0)
opt.append((x, (i - 1, j - 1)))
mi = min(opt)
dist[i, j] = mi[0]
pred[i, j] = mi[1]
return dist[len(mot1) - 1, len(mot2) - 1]
_edit_distance("abdc", "cbda")
2
_edit_distance((0, 1, 2, 3), (0, 2, 1, 3))
2
from math import log
def _is_rotation(perm):
t = tuple(perm)
c = list(range(len(perm)))
for i in range(len(c)):
for k in range(len(c)):
c[k] = (k + i) % len(c)
if t == tuple(c):
return True
return False
def _relu(x, origin=0):
return origin if x < origin else x
def compute_features(shape, perm):
total = numpy.prod(numpy.array(shape, dtype=numpy.int64))
begin = 1
dbegin = 0
for i, p in enumerate(perm):
if p != i:
break
dbegin += 1
begin *= shape[i]
end = 1
dend = 0
for i in range(len(perm)-1, -1, -1):
if perm[i] != i:
break
dend += 1
end *= shape[i]
dis_cont = 0
for i in range(1, len(shape)):
if perm[i] != perm[i-1] + 1:
dis_cont += 1
middle = max(1, int(total / (end * begin)))
feat = dict(size=total, begin=begin, end=end, middle=middle,
dim=len(shape), discont=dis_cont)
for c in [16, 32]:
feat["end%d" % c] = _relu(end, c)
keys = list(feat)
for k in keys:
if k in {'dim', 'cpu', 'size'}:
continue
feat['r%s' % k] = float(feat[k] / total)
for c in [2, 4, 8, 16, 32, 64]:
feat["iend%d" % c] = float(end >= c)
feat["ibegin%d" % c] = float(begin >= c)
# feat['CST'] = 1
feat['CST_'] = -1
feat['dbegin'] = - dbegin
feat['dend'] = - dend
keys = list(feat)
for k in keys:
if k.startswith('end') or k.startswith('begin'):
feat[k] = - feat[k]
elif k.startswith('rend') or k.startswith('rbegin'):
feat[k] = - feat[k]
elif k.startswith('iend') or k.startswith('ibegin'):
feat[k] = - feat[k]
elif k == "rdiscont":
feat[k] = - feat[k]
idp = list(range(len(perm)))
feat["rot"] = -1 if _is_rotation(perm) else 0
feat["rev"] = 1 if perm == tuple(idp[::-1]) else 0
feat["edit"] = _edit_distance(idp, perm)
feat["redit"] = feat["edit"] / len(idp)
return feat
compute_features((3, 5, 7), (0, 1, 2))
{'size': 105,
'begin': -105,
'end': -105,
'middle': 1,
'dim': 3,
'discont': 0,
'end16': -105,
'end32': -105,
'rbegin': -1.0,
'rend': -1.0,
'rmiddle': 0.009523809523809525,
'rdiscont': -0.0,
'rend16': -1.0,
'rend32': -1.0,
'iend2': -1.0,
'ibegin2': -1.0,
'iend4': -1.0,
'ibegin4': -1.0,
'iend8': -1.0,
'ibegin8': -1.0,
'iend16': -1.0,
'ibegin16': -1.0,
'iend32': -1.0,
'ibegin32': -1.0,
'iend64': -1.0,
'ibegin64': -1.0,
'CST_': -1,
'dbegin': -3,
'dend': -3,
'rot': -1,
'rev': 0,
'edit': 0,
'redit': 0.0}
compute_features((3, 5, 7), (2, 1, 0))
{'size': 105,
'begin': -1,
'end': -1,
'middle': 105,
'dim': 3,
'discont': 2,
'end16': -16,
'end32': -32,
'rbegin': -0.009523809523809525,
'rend': -0.009523809523809525,
'rmiddle': 1.0,
'rdiscont': -0.01904761904761905,
'rend16': -0.1523809523809524,
'rend32': -0.3047619047619048,
'iend2': -0.0,
'ibegin2': -0.0,
'iend4': -0.0,
'ibegin4': -0.0,
'iend8': -0.0,
'ibegin8': -0.0,
'iend16': -0.0,
'ibegin16': -0.0,
'iend32': -0.0,
'ibegin32': -0.0,
'iend64': -0.0,
'ibegin64': -0.0,
'CST_': -1,
'dbegin': 0,
'dend': 0,
'rot': 0,
'rev': 1,
'edit': 2,
'redit': 0.6666666666666666}
compute_features((3, 5, 7), (1, 2, 0))
{'size': 105,
'begin': -1,
'end': -1,
'middle': 105,
'dim': 3,
'discont': 1,
'end16': -16,
'end32': -32,
'rbegin': -0.009523809523809525,
'rend': -0.009523809523809525,
'rmiddle': 1.0,
'rdiscont': -0.009523809523809525,
'rend16': -0.1523809523809524,
'rend32': -0.3047619047619048,
'iend2': -0.0,
'ibegin2': -0.0,
'iend4': -0.0,
'ibegin4': -0.0,
'iend8': -0.0,
'ibegin8': -0.0,
'iend16': -0.0,
'ibegin16': -0.0,
'iend32': -0.0,
'ibegin32': -0.0,
'iend64': -0.0,
'ibegin64': -0.0,
'CST_': -1,
'dbegin': 0,
'dend': 0,
'rot': -1,
'rev': 0,
'edit': 2,
'redit': 0.6666666666666666}
Computing the features for all simulations#
def compute_features_dataframe(df):
def merge(row):
feat = compute_features(row['shape'], row['perm'])
feat['yt'] = row['average']
feat['yr'] = row['ratio']
return feat
rows = []
for i in tqdm(range(df.shape[0])):
rows.append(dict(shape=df.loc[i, "shape"], perm=df.loc[i, "perm"],
average=df.loc[i, "average"], ratio=df.loc[i, "ratio"]))
obs = []
for row in tqdm(rows):
obs.append(merge(row))
return DataFrame(obs)
fdata = compute_features_dataframe(data)
col_sort = list(sorted(fdata.columns))
fdata = fdata[col_sort]
fdata.tail()
100%|██████████| 132/132 [00:00<00:00, 9459.22it/s]
100%|██████████| 132/132 [00:00<00:00, 3601.95it/s]
CST_ | begin | dbegin | dend | dim | discont | edit | end | end16 | end32 | ... | redit | rend | rend16 | rend32 | rev | rmiddle | rot | size | yr | yt | |
---|---|---|---|---|---|---|---|---|---|---|---|---|---|---|---|---|---|---|---|---|---|
127 | -1 | -3 | -1 | 0 | 4 | 3 | 2 | -1 | -16 | -32 | ... | 0.50 | -9.331422e-07 | -0.000015 | -0.00003 | 0 | 0.333333 | 0 | 1071648 | 2.790823 | 0.010339 |
128 | -1 | -1 | 0 | 0 | 4 | 2 | 4 | -1 | -16 | -32 | ... | 1.00 | -9.331422e-07 | -0.000015 | -0.00003 | 0 | 1.000000 | 0 | 1071648 | 2.882191 | 0.010677 |
129 | -1 | -1 | 0 | 0 | 4 | 3 | 4 | -1 | -16 | -32 | ... | 1.00 | -9.331422e-07 | -0.000015 | -0.00003 | 1 | 1.000000 | 0 | 1071648 | 3.352770 | 0.012421 |
130 | -1 | -1 | 0 | 0 | 4 | 2 | 4 | -1 | -16 | -32 | ... | 1.00 | -9.331422e-07 | -0.000015 | -0.00003 | 0 | 1.000000 | 0 | 1071648 | 3.625680 | 0.013432 |
131 | -1 | -1 | 0 | 0 | 4 | 3 | 3 | -1 | -16 | -32 | ... | 0.75 | -9.331422e-07 | -0.000015 | -0.00003 | 0 | 1.000000 | 0 | 1071648 | 3.935483 | 0.014579 |
5 rows × 35 columns
correlations#
fdata.corr()
CST_ | begin | dbegin | dend | dim | discont | edit | end | end16 | end32 | ... | redit | rend | rend16 | rend32 | rev | rmiddle | rot | size | yr | yt | |
---|---|---|---|---|---|---|---|---|---|---|---|---|---|---|---|---|---|---|---|---|---|
CST_ | NaN | NaN | NaN | NaN | NaN | NaN | NaN | NaN | NaN | NaN | ... | NaN | NaN | NaN | NaN | NaN | NaN | NaN | NaN | NaN | NaN |
begin | NaN | 1.000000 | 0.596816 | 0.596414 | 0.014118 | 0.404952 | 0.405175 | 0.999998 | 0.999998 | 0.999998 | ... | 0.418022 | 0.681573 | 0.681573 | 0.681573 | 0.038216 | 0.256349 | 0.325594 | -0.133581 | 0.127658 | -0.008816 |
dbegin | NaN | 0.596816 | 1.000000 | 0.676899 | 0.077162 | 0.486887 | 0.669598 | 0.596384 | 0.596374 | 0.596363 | ... | 0.690333 | 0.831887 | 0.831895 | 0.831903 | 0.111636 | 0.605990 | 0.298090 | 0.016411 | 0.291318 | 0.139951 |
dend | NaN | 0.596414 | 0.676899 | 1.000000 | 0.077162 | 0.486887 | 0.669598 | 0.596936 | 0.596907 | 0.596881 | ... | 0.690333 | 0.833059 | 0.832975 | 0.832924 | 0.111636 | 0.623582 | 0.298090 | 0.016411 | 0.305489 | 0.155098 |
dim | NaN | 0.014118 | 0.077162 | 0.077162 | 1.000000 | 0.305320 | 0.272614 | 0.014153 | 0.014145 | 0.014135 | ... | 0.115902 | 0.160407 | 0.160417 | 0.160414 | -0.160357 | 0.106693 | 0.240946 | 0.212685 | 0.138961 | 0.192305 |
discont | NaN | 0.404952 | 0.486887 | 0.486887 | 0.305320 | 1.000000 | 0.531254 | 0.404971 | 0.404961 | 0.404948 | ... | 0.504206 | 0.594219 | 0.594226 | 0.594223 | 0.150144 | 0.225854 | 0.823937 | 0.064937 | 0.388140 | 0.203342 |
edit | NaN | 0.405175 | 0.669598 | 0.669598 | 0.272614 | 0.531254 | 1.000000 | 0.405223 | 0.405204 | 0.405189 | ... | 0.984655 | 0.594688 | 0.594639 | 0.594619 | 0.208568 | 0.652532 | 0.338994 | 0.057981 | 0.464225 | 0.283262 |
end | NaN | 0.999998 | 0.596384 | 0.596936 | 0.014153 | 0.404971 | 0.405223 | 1.000000 | 1.000000 | 1.000000 | ... | 0.418062 | 0.681565 | 0.681565 | 0.681565 | 0.038236 | 0.256479 | 0.325559 | -0.133665 | 0.127730 | -0.008844 |
end16 | NaN | 0.999998 | 0.596374 | 0.596907 | 0.014145 | 0.404961 | 0.405204 | 1.000000 | 1.000000 | 1.000000 | ... | 0.418044 | 0.681550 | 0.681550 | 0.681550 | 0.038231 | 0.256451 | 0.325557 | -0.133671 | 0.127716 | -0.008852 |
end32 | NaN | 0.999998 | 0.596363 | 0.596881 | 0.014135 | 0.404948 | 0.405189 | 1.000000 | 1.000000 | 1.000000 | ... | 0.418029 | 0.681533 | 0.681533 | 0.681533 | 0.038228 | 0.256430 | 0.325552 | -0.133677 | 0.127707 | -0.008859 |
ibegin16 | NaN | 0.488586 | 0.854056 | 0.553792 | 0.160800 | 0.476225 | 0.528132 | 0.487938 | 0.487930 | 0.487919 | ... | 0.533981 | 0.715870 | 0.715889 | 0.715901 | 0.078215 | 0.522399 | 0.283800 | 0.037462 | 0.254352 | 0.136416 |
ibegin2 | NaN | 0.297779 | 0.792225 | 0.326418 | 0.080033 | 0.230393 | 0.539082 | 0.297285 | 0.297281 | 0.297277 | ... | 0.548605 | 0.436111 | 0.436126 | 0.436148 | 0.128338 | 0.685605 | 0.049586 | -0.027198 | 0.324851 | 0.109929 |
ibegin32 | NaN | 0.488586 | 0.854056 | 0.553792 | 0.160800 | 0.476225 | 0.528132 | 0.487938 | 0.487930 | 0.487919 | ... | 0.533981 | 0.715870 | 0.715889 | 0.715901 | 0.078215 | 0.522399 | 0.283800 | 0.037462 | 0.254352 | 0.136416 |
ibegin4 | NaN | 0.420023 | 0.814178 | 0.474232 | 0.114432 | 0.388689 | 0.517951 | 0.419433 | 0.419424 | 0.419415 | ... | 0.528284 | 0.615385 | 0.615471 | 0.615584 | 0.090985 | 0.594774 | 0.207651 | 0.116460 | 0.278810 | 0.179014 |
ibegin64 | NaN | 0.510659 | 0.869357 | 0.586430 | 0.083333 | 0.488512 | 0.533376 | 0.509999 | 0.509990 | 0.509979 | ... | 0.555529 | 0.748243 | 0.748253 | 0.748257 | 0.074833 | 0.501069 | 0.307207 | 0.017724 | 0.243128 | 0.124459 |
ibegin8 | NaN | 0.420023 | 0.814178 | 0.474232 | 0.114432 | 0.388689 | 0.517951 | 0.419433 | 0.419424 | 0.419415 | ... | 0.528284 | 0.615385 | 0.615471 | 0.615584 | 0.090985 | 0.594774 | 0.207651 | 0.116460 | 0.278810 | 0.179014 |
iend16 | NaN | 0.405858 | 0.452474 | 0.807989 | 0.181503 | 0.383654 | 0.517311 | 0.406614 | 0.406575 | 0.406542 | ... | 0.513917 | 0.597165 | 0.597061 | 0.597069 | 0.094032 | 0.619928 | 0.191751 | 0.125689 | 0.307633 | 0.182267 |
iend2 | NaN | 0.297323 | 0.326418 | 0.792225 | 0.080033 | 0.230393 | 0.539082 | 0.297930 | 0.297895 | 0.297872 | ... | 0.548605 | 0.437541 | 0.437398 | 0.437338 | 0.128338 | 0.724562 | 0.049586 | -0.027198 | 0.337071 | 0.146321 |
iend32 | NaN | 0.468224 | 0.524298 | 0.841277 | 0.233408 | 0.465593 | 0.524167 | 0.469061 | 0.469029 | 0.468993 | ... | 0.514805 | 0.688714 | 0.688640 | 0.688553 | 0.081511 | 0.544599 | 0.262448 | 0.049643 | 0.261930 | 0.139099 |
iend4 | NaN | 0.360597 | 0.400119 | 0.792963 | 0.141421 | 0.321878 | 0.519634 | 0.361290 | 0.361251 | 0.361222 | ... | 0.521120 | 0.530594 | 0.530467 | 0.530439 | 0.105830 | 0.673384 | 0.136300 | -0.039355 | 0.351151 | 0.127468 |
iend64 | NaN | 0.487959 | 0.553792 | 0.854056 | 0.160800 | 0.476225 | 0.528132 | 0.488816 | 0.488786 | 0.488752 | ... | 0.533981 | 0.717677 | 0.717612 | 0.717534 | 0.078215 | 0.523746 | 0.283800 | 0.030938 | 0.255567 | 0.127728 |
iend8 | NaN | 0.405858 | 0.452474 | 0.807989 | 0.181503 | 0.383654 | 0.517311 | 0.406614 | 0.406575 | 0.406542 | ... | 0.513917 | 0.597165 | 0.597061 | 0.597069 | 0.094032 | 0.619928 | 0.191751 | 0.125689 | 0.307633 | 0.182267 |
middle | NaN | 0.126896 | 0.303868 | 0.319057 | 0.178317 | 0.152095 | 0.377874 | 0.126960 | 0.126947 | 0.126937 | ... | 0.355980 | 0.186472 | 0.186669 | 0.186918 | 0.052991 | 0.467981 | 0.000903 | 0.728990 | -0.008120 | 0.821357 |
rbegin | NaN | 0.681576 | 0.832794 | 0.831933 | 0.160296 | 0.594171 | 0.594568 | 0.681564 | 0.681549 | 0.681532 | ... | 0.613417 | 0.999992 | 0.999993 | 0.999993 | 0.056108 | 0.376357 | 0.477649 | 0.034328 | 0.187411 | 0.095471 |
rdiscont | NaN | -0.132163 | -0.158903 | -0.158903 | -0.077379 | -0.320270 | -0.168278 | -0.132191 | -0.132195 | -0.132192 | ... | -0.163660 | -0.193464 | -0.193106 | -0.192632 | -0.054527 | -0.001602 | -0.265672 | 0.551880 | 0.004517 | 0.386893 |
redit | NaN | 0.418022 | 0.690333 | 0.690333 | 0.115902 | 0.504206 | 0.984655 | 0.418062 | 0.418044 | 0.418029 | ... | 1.000000 | 0.613517 | 0.613466 | 0.613446 | 0.244134 | 0.655658 | 0.317106 | 0.024651 | 0.450928 | 0.256097 |
rend | NaN | 0.681573 | 0.831887 | 0.833059 | 0.160407 | 0.594219 | 0.594688 | 0.681565 | 0.681550 | 0.681533 | ... | 0.613517 | 1.000000 | 1.000000 | 0.999999 | 0.056153 | 0.376658 | 0.477579 | 0.034412 | 0.187551 | 0.095557 |
rend16 | NaN | 0.681573 | 0.831895 | 0.832975 | 0.160417 | 0.594226 | 0.594639 | 0.681565 | 0.681550 | 0.681533 | ... | 0.613466 | 1.000000 | 1.000000 | 1.000000 | 0.056129 | 0.376574 | 0.477613 | 0.034679 | 0.187559 | 0.095755 |
rend32 | NaN | 0.681573 | 0.831903 | 0.832924 | 0.160414 | 0.594223 | 0.594619 | 0.681565 | 0.681550 | 0.681533 | ... | 0.613446 | 0.999999 | 1.000000 | 1.000000 | 0.056116 | 0.376557 | 0.477630 | 0.035005 | 0.187607 | 0.095996 |
rev | NaN | 0.038216 | 0.111636 | 0.111636 | -0.160357 | 0.150144 | 0.208568 | 0.038236 | 0.038231 | 0.038228 | ... | 0.244134 | 0.056153 | 0.056129 | 0.056116 | 1.000000 | 0.180470 | 0.117200 | -0.034106 | 0.218387 | 0.094260 |
rmiddle | NaN | 0.256349 | 0.605990 | 0.623582 | 0.106693 | 0.225854 | 0.652532 | 0.256479 | 0.256451 | 0.256430 | ... | 0.655658 | 0.376658 | 0.376574 | 0.376557 | 0.180470 | 1.000000 | -0.064351 | -0.013771 | 0.468925 | 0.195497 |
rot | NaN | 0.325594 | 0.298090 | 0.298090 | 0.240946 | 0.823937 | 0.338994 | 0.325559 | 0.325557 | 0.325552 | ... | 0.317106 | 0.477579 | 0.477613 | 0.477630 | 0.117200 | -0.064351 | 1.000000 | 0.051246 | 0.243294 | 0.126195 |
size | NaN | -0.133581 | 0.016411 | 0.016411 | 0.212685 | 0.064937 | 0.057981 | -0.133665 | -0.133671 | -0.133677 | ... | 0.024651 | 0.034412 | 0.034679 | 0.035005 | -0.034106 | -0.013771 | 0.051246 | 1.000000 | -0.236289 | 0.805926 |
yr | NaN | 0.127658 | 0.291318 | 0.305489 | 0.138961 | 0.388140 | 0.464225 | 0.127730 | 0.127716 | 0.127707 | ... | 0.450928 | 0.187551 | 0.187559 | 0.187607 | 0.218387 | 0.468925 | 0.243294 | -0.236289 | 1.000000 | -0.013907 |
yt | NaN | -0.008816 | 0.139951 | 0.155098 | 0.192305 | 0.203342 | 0.283262 | -0.008844 | -0.008852 | -0.008859 | ... | 0.256097 | 0.095557 | 0.095755 | 0.095996 | 0.094260 | 0.195497 | 0.126195 | 0.805926 | -0.013907 | 1.000000 |
35 rows × 35 columns
fdata.corr()['yt']
CST_ NaN
begin -0.008816
dbegin 0.139951
dend 0.155098
dim 0.192305
discont 0.203342
edit 0.283262
end -0.008844
end16 -0.008852
end32 -0.008859
ibegin16 0.136416
ibegin2 0.109929
ibegin32 0.136416
ibegin4 0.179014
ibegin64 0.124459
ibegin8 0.179014
iend16 0.182267
iend2 0.146321
iend32 0.139099
iend4 0.127468
iend64 0.127728
iend8 0.182267
middle 0.821357
rbegin 0.095471
rdiscont 0.386893
redit 0.256097
rend 0.095557
rend16 0.095755
rend32 0.095996
rev 0.094260
rmiddle 0.195497
rot 0.126195
size 0.805926
yr -0.013907
yt 1.000000
Name: yt, dtype: float64
We check the sign of the correlations of all features with yt. If it
is positive, increasing the feature increases the processing time. We
try to get only positive correlations. end is the flattened last
dimensions left unchanged by the permutation. The bigger it is, the
faster the transposition is. That’s why the function computing all
features multiplies this number by -1
to get a feature positively
correlated to the processing time. end16 is equal to end when
end<-16
and -16
when end>=-16
. This is a simplification of
the cost of moving data from memory to cache L1. This cost is linear
when the data to move is big enough, but almost constant for small
chunks.
Linear regression#
We choose a linear regression because the prediction are not limited. The training set does not include all configuration and surely does not include all possible high value the model may have to predict.
The goal is not necessarily to predict the fastest permutation but to predict the processing time as the goal is to find the best combination of transpositions in a ONNX graph (einsum). The final goal is to predict which graphs optimizes a series of transpositions.
The target could be the processing time or the logarithm of this time. However, making mistakes on small times is not an issue but errors on high processing time is not a good thing.
We could also try to predict a ratio transposition time /copy time but it still gives more important to small matrix size.
Many variables are correlated. Variables should be selected.
Dataset#
X = fdata.drop(["yt", "yr"], axis=1)
x_names = list(X.columns)
yt = fdata['yt'] * 1000
numpy.mean(yt)
1.8809171132996723
Simple model#
from sklearn.linear_model import LinearRegression
from sklearn.preprocessing import StandardScaler
from sklearn.pipeline import make_pipeline
from sklearn.metrics import r2_score, mean_absolute_error
pipe = make_pipeline(StandardScaler(with_mean=False), LinearRegression(fit_intercept=False))
pipe.fit(X, yt)
model = pipe.steps[1][1]
coef = {k: v for k, v in zip(X.columns, model.coef_)}
coef['name'] = 'reg'
coef['intercept_'] = model.intercept_
pred = numpy.maximum(pipe.predict(X), 0)
coef['r2'] = r2_score(yt, pred)
coef['mae'] = mean_absolute_error(yt, pred)
coef['model'] = pipe
coefs = [coef]
coef["r2"], coef['mae']
(0.8157414076410756, 0.6368865305095469)
df = DataFrame([(k, v) for k, v in coef.items() if k not in {'name', 'model'}],
columns=["feature", "value"]).set_index("feature")
df.plot(kind="bar", figsize=(14, 2));
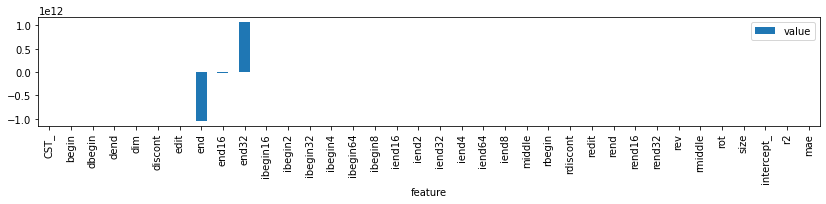
df
value | |
---|---|
feature | |
CST_ | -3.076618e+08 |
begin | -2.941725e+01 |
dbegin | -1.854147e-01 |
dend | -9.638954e-02 |
dim | -1.037599e-01 |
discont | 5.204404e-01 |
edit | 3.582481e-01 |
end | -1.046584e+12 |
end16 | -2.278042e+10 |
end32 | 1.069321e+12 |
ibegin16 | -3.713466e+00 |
ibegin2 | 1.439716e-02 |
ibegin32 | 3.784367e+00 |
ibegin4 | -6.813416e+00 |
ibegin64 | -7.576102e-02 |
ibegin8 | 6.927856e+00 |
iend16 | 2.028144e+07 |
iend2 | 8.225773e+06 |
iend32 | 4.322857e+07 |
iend4 | 1.097274e+07 |
iend64 | 1.996315e-01 |
iend8 | 2.028143e+07 |
middle | 1.541218e+00 |
rbegin | 4.940619e+01 |
rdiscont | 7.614642e-01 |
redit | 8.622710e-02 |
rend | 6.615750e+02 |
rend16 | 3.459172e+02 |
rend32 | -1.057057e+03 |
rev | 1.537206e-01 |
rmiddle | -4.563712e-01 |
rot | 7.771901e-02 |
size | 1.295707e+00 |
intercept_ | 0.000000e+00 |
r2 | 8.157414e-01 |
mae | 6.368865e-01 |
Coefficients associated to features end, end16 are almost opposed and it would better to get a model which keeps only one.
Quantile Regression#
from mlinsights.mlmodel import QuantileLinearRegression
pipe = make_pipeline(StandardScaler(with_mean=False), QuantileLinearRegression(fit_intercept=False))
pipe.fit(X, yt)
model = pipe.steps[1][1]
coef = {k: v for k, v in zip(X.columns, model.coef_)}
coef['name'] = 'med'
coef['intercept_'] = model.intercept_
pred = numpy.maximum(pipe.predict(X), 0)
coef['r2'] = r2_score(yt, pred)
coef['mae'] = mean_absolute_error(yt, pred)
coef['model'] = pipe
coefs.append(coef)
coef["r2"], coef['mae']
(0.7924498414927943, 0.5679387557069854)
DataFrame(coef.items(), columns=["feature", "value"]).set_index("feature")
value | |
---|---|
feature | |
CST_ | 1433409.249051 |
begin | 27.13405 |
dbegin | 0.07931 |
dend | 0.087576 |
dim | 0.006919 |
discont | 0.413378 |
edit | 0.186032 |
end | 4876069525.422424 |
end16 | 106134745.367844 |
end32 | -4982003112.711292 |
ibegin16 | 0.129918 |
ibegin2 | -0.069604 |
ibegin32 | -0.221099 |
ibegin4 | -0.045585 |
ibegin64 | -0.1085 |
ibegin8 | 0.073031 |
iend16 | -94492.918693 |
iend2 | -38324.37475 |
iend32 | -201401.795017 |
iend4 | -51122.392443 |
iend64 | 0.15928 |
iend8 | -94492.881923 |
middle | 1.588707 |
rbegin | 36.958438 |
rdiscont | 0.375421 |
redit | 0.071189 |
rend | 4424.263222 |
rend16 | -7664.018684 |
rend32 | 3202.681647 |
rev | 0.08288 |
rmiddle | -0.207068 |
rot | -0.095643 |
size | 0.938597 |
name | med |
intercept_ | 0 |
r2 | 0.79245 |
mae | 0.567939 |
model | (StandardScaler(with_mean=False), QuantileLine... |
Lasso#
To select features.
from sklearn.linear_model import Lasso
scores = []
models = []
for a in tqdm([0.001, 0.01, 0.1, 0.2, 0.3, 0.4, 0.5, 0.6, 0.7, 0.8, 0.9, 1., 2.]):
alpha = a * 1.
pipe = make_pipeline(
StandardScaler(with_mean=False),
Lasso(alpha=alpha, fit_intercept=False, max_iter=5000))
pipe.fit(X, yt)
pred = numpy.maximum(pipe.predict(X), 0)
model = pipe.steps[1][1]
scores.append(dict(r2=r2_score(yt, pred), mae=mean_absolute_error(yt, pred),
alpha=alpha, null=(numpy.abs(model.coef_) < 1e-6).sum(),
n=len(model.coef_)))
models.append(pipe)
if alpha >= 0.01 and alpha <= 0.2:
coef = {k: v for k, v in zip(X.columns, pipe.steps[1][1].coef_)}
coef['name'] = "Lasso-%f" % alpha
coef['model'] = pipe
coef['r2'] = r2_score(yt, pred)
coef['mae'] = mean_absolute_error(yt, pred)
coefs.append(coef)
DataFrame(scores)
100%|██████████| 13/13 [00:00<00:00, 69.97it/s]
r2 | mae | alpha | null | n | |
---|---|---|---|---|---|
0 | 0.809704 | 0.629480 | 0.001 | 4 | 33 |
1 | 0.807546 | 0.629886 | 0.010 | 10 | 33 |
2 | 0.782541 | 0.676499 | 0.100 | 23 | 33 |
3 | 0.766911 | 0.680344 | 0.200 | 28 | 33 |
4 | 0.751546 | 0.703684 | 0.300 | 29 | 33 |
5 | 0.738223 | 0.742962 | 0.400 | 30 | 33 |
6 | 0.730937 | 0.735958 | 0.500 | 31 | 33 |
7 | 0.718437 | 0.758143 | 0.600 | 30 | 33 |
8 | 0.701329 | 0.800503 | 0.700 | 30 | 33 |
9 | 0.681590 | 0.848549 | 0.800 | 30 | 33 |
10 | 0.659218 | 0.898770 | 0.900 | 30 | 33 |
11 | 0.634218 | 0.949493 | 1.000 | 30 | 33 |
12 | 0.239413 | 1.600542 | 2.000 | 30 | 33 |
coef = {k: v for k, v in zip(X.columns, models[1].steps[1][1].coef_)}
df = DataFrame(coef.items(), columns=["feature", "value"]).set_index("feature")
df.plot(kind="bar", figsize=(14, 2), title="alpha=%f" % scores[1]["alpha"]);
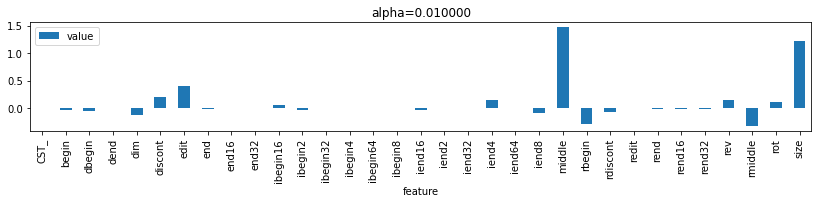
coef = {k: v for k, v in zip(X.columns, models[2].steps[1][1].coef_)}
df = DataFrame(coef.items(), columns=["feature", "value"]).set_index("feature")
df.plot(kind="bar", figsize=(14, 2), title="alpha=%f" % scores[2]["alpha"]);
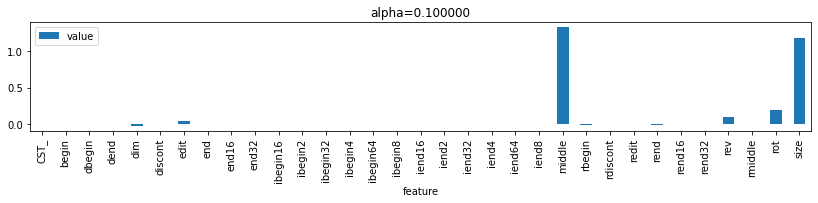
Linear regression with positive weights#
pipe = make_pipeline(StandardScaler(with_mean=False), LinearRegression(positive=True, fit_intercept=False))
pipe.fit(X, yt)
model = pipe.steps[1][1]
coef = {k: v for k, v in zip(X.columns, model.coef_)}
coef['name'] = 'pos'
coef['intercept_'] = model.intercept_
pred = numpy.maximum(pipe.predict(X), 0)
coef['r2'] = r2_score(yt, pred)
coef['mae'] = mean_absolute_error(yt, pred)
coef['model'] = pipe
coefs.append(coef)
coef["r2"], coef['mae']
(0.7905447080626958, 0.6768663007518693)
coef = {k: v for k, v in zip(X.columns, pipe.steps[1][1].coef_)}
df = DataFrame(coef.items(), columns=["feature", "value"]).set_index("feature")
df.plot(kind="bar", figsize=(14, 2), title="positive");
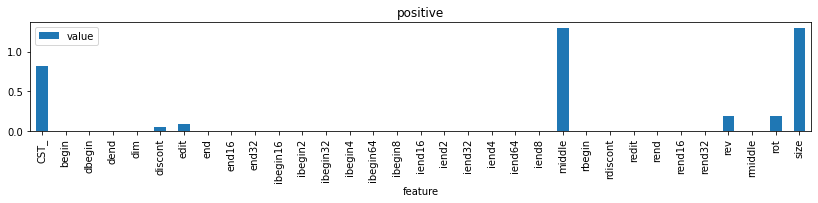
Quantile regression with positive weights#
pipe = make_pipeline(StandardScaler(with_mean=False), QuantileLinearRegression(positive=True, fit_intercept=False))
pipe.fit(X, yt)
model = pipe.steps[1][1]
coef = {k: v for k, v in zip(X.columns, model.coef_)}
coef['name'] = 'medpos'
coef['intercept_'] = model.intercept_
pred = numpy.maximum(pipe.predict(X), 0)
coef['r2'] = r2_score(yt, pred)
coef['mae'] = mean_absolute_error(yt, pred)
coef['model'] = pipe
coefs.append(coef)
coef["r2"], coef['mae']
(0.752689515971656, 0.6468340444504788)
coef = {k: v for k, v in zip(X.columns, pipe.steps[1][1].coef_)}
df = DataFrame(coef.items(), columns=["feature", "value"]).set_index("feature")
df.plot(kind="bar", figsize=(14, 2), title="positive");
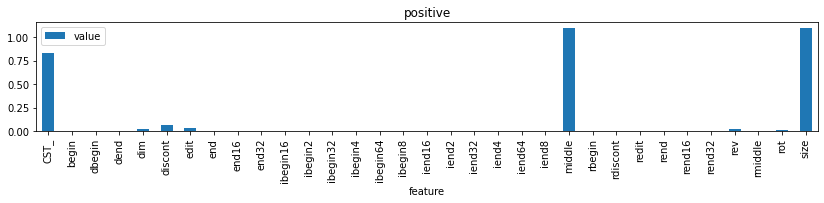
Summary#
dfcoef = DataFrame(coefs)
dfcoef[::-1].T
6 | 5 | 4 | 3 | 2 | 1 | 0 | |
---|---|---|---|---|---|---|---|
CST_ | 0.829482 | 0.821048 | 0.0 | 0.0 | 0.0 | 1433409.249051 | -307661768.128088 |
begin | 0.0 | 0.0 | -0.0 | -0.0 | -0.03443 | 27.13405 | -29.417247 |
dbegin | 0.0 | 0.0 | -0.0 | -0.0 | -0.044705 | 0.07931 | -0.185415 |
dend | 0.0 | 0.0 | -0.0 | -0.0 | -0.0 | 0.087576 | -0.09639 |
dim | 0.023846 | 0.0 | -0.014763 | -0.030446 | -0.120949 | 0.006919 | -0.10376 |
discont | 0.060636 | 0.056297 | 0.0 | 0.0 | 0.210421 | 0.413378 | 0.52044 |
edit | 0.03823 | 0.094856 | 0.0 | 0.0418 | 0.396052 | 0.186032 | 0.358248 |
end | 0.0 | 0.0 | -0.0 | -0.0 | -0.007053 | 4876069525.422424 | -1046583604803.358887 |
end16 | 0.0 | 0.0 | -0.0 | -0.0 | -0.000036 | 106134745.367844 | -22780416305.902706 |
end32 | 0.0 | 0.0 | -0.0 | -0.0 | -0.00004 | -4982003112.711292 | 1069320839370.567505 |
ibegin16 | 0.0 | 0.0 | -0.0 | -0.0 | 0.066669 | 0.129918 | -3.713466 |
ibegin2 | 0.0 | 0.0 | -0.0 | -0.0 | -0.02181 | -0.069604 | 0.014397 |
ibegin32 | 0.0 | 0.0 | -0.0 | -0.0 | 0.0 | -0.221099 | 3.784367 |
ibegin4 | 0.0 | 0.0 | -0.0 | -0.0 | 0.0 | -0.045585 | -6.813416 |
ibegin64 | 0.0 | 0.0 | -0.0 | -0.0 | 0.0 | -0.1085 | -0.075761 |
ibegin8 | 0.0 | 0.0 | -0.0 | -0.0 | 0.0 | 0.073031 | 6.927856 |
iend16 | 0.0 | 0.0 | -0.0 | -0.0 | -0.022416 | -94492.918693 | 20281439.108194 |
iend2 | 0.0 | 0.0 | 0.0 | -0.0 | 0.0 | -38324.37475 | 8225773.255917 |
iend32 | 0.0 | 0.0 | -0.0 | -0.0 | -0.0 | -201401.795017 | 43228573.054944 |
iend4 | 0.0 | 0.0 | -0.0 | -0.0 | 0.151081 | -51122.392443 | 10972737.091606 |
iend64 | 0.0 | 0.0 | -0.0 | -0.0 | -0.0 | 0.15928 | 0.199631 |
iend8 | 0.0 | 0.0 | -0.0 | -0.0 | -0.08907 | -94492.881923 | 20281426.580972 |
middle | 1.101543 | 1.30347 | 1.290699 | 1.325916 | 1.466733 | 1.588707 | 1.541218 |
rbegin | 0.0 | 0.0 | -0.0 | -0.020369 | -0.28295 | 36.958438 | 49.406192 |
rdiscont | 0.0 | 0.0 | 0.0 | -0.0 | -0.066385 | 0.375421 | 0.761464 |
redit | 0.0 | 0.0 | 0.0 | 0.0 | 0.0 | 0.071189 | 0.086227 |
rend | 0.0 | 0.0 | -0.0 | -0.007655 | -0.007593 | 4424.263222 | 661.575013 |
rend16 | 0.0 | 0.0 | -0.0 | -0.003393 | -0.010514 | -7664.018684 | 345.917179 |
rend32 | 0.0 | 0.0 | -0.0 | -0.005349 | -0.013172 | 3202.681647 | -1057.05651 |
rev | 0.026757 | 0.189909 | 0.013992 | 0.097585 | 0.142791 | 0.08288 | 0.153721 |
rmiddle | 0.0 | 0.0 | -0.0 | -0.0 | -0.324716 | -0.207068 | -0.456371 |
rot | 0.009222 | 0.185687 | 0.108468 | 0.197021 | 0.108146 | -0.095643 | 0.077719 |
size | 1.100532 | 1.300222 | 1.099463 | 1.183553 | 1.22329 | 0.938597 | 1.295707 |
name | medpos | pos | Lasso-0.200000 | Lasso-0.100000 | Lasso-0.010000 | med | reg |
intercept_ | 0.0 | 0.0 | NaN | NaN | NaN | 0.0 | 0.0 |
r2 | 0.75269 | 0.790545 | 0.766911 | 0.782541 | 0.807546 | 0.79245 | 0.815741 |
mae | 0.646834 | 0.676866 | 0.680344 | 0.676499 | 0.629886 | 0.567939 | 0.636887 |
model | (StandardScaler(with_mean=False), QuantileLine... | (StandardScaler(with_mean=False), LinearRegres... | (StandardScaler(with_mean=False), Lasso(alpha=... | (StandardScaler(with_mean=False), Lasso(alpha=... | (StandardScaler(with_mean=False), Lasso(alpha=... | (StandardScaler(with_mean=False), QuantileLine... | (StandardScaler(with_mean=False), LinearRegres... |
dfcoef[["name", "r2", "mae"]].set_index('name').plot(kind="bar", title="performance accross models");
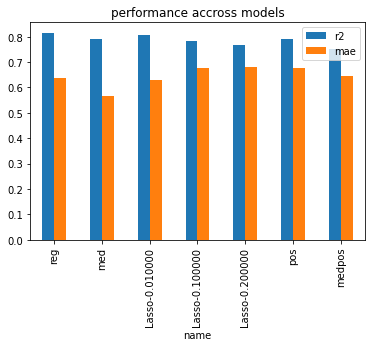
import matplotlib.pyplot as plt
dfp = dfcoef.drop(['name', 'model'], axis=1).T.drop([0, 1], axis=1).copy()
dfp.columns = dfcoef['name'][2:]
ax = dfp.plot(figsize=(14, 4), kind="line")
ax.set_xticks(numpy.arange(0, dfp.shape[0]))
ax.set_xticklabels(dfp.index)
plt.setp(ax.get_xticklabels(), rotation=45, horizontalalignment='right');
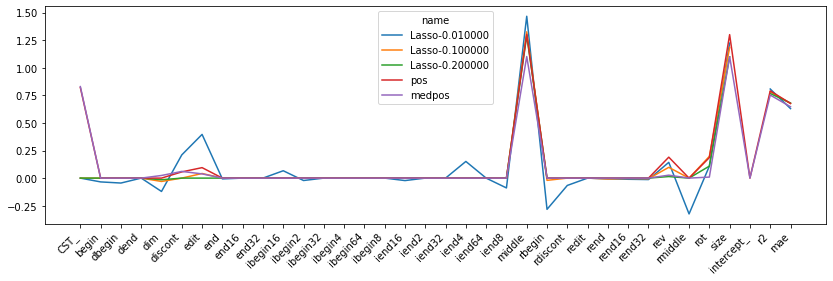
Investigation#
data_err = data.drop(["context_size", "repeat"], axis=1).copy()
data_err['predict'] = numpy.maximum(coefs[0]['model'].predict(X), 0) / 1000
data_err['err'] = (data_err['predict'] - data_err['average'])
data_err['abserr'] = numpy.abs(data_err['predict'] - data_err['average'])
data_err['rel'] = (data_err['predict'] - data_err['average']) / data_err['average']
s = data_err.sort_values('abserr')
pandas.concat([s.head(n=10), s.tail(n=10)])
average | deviation | min_exec | max_exec | number | perm | shape | ratio | dim | predict | err | abserr | rel | |
---|---|---|---|---|---|---|---|---|---|---|---|---|---|
28 | 0.000113 | 0.000029 | 0.000061 | 0.000141 | 50 | (1, 0, 2) | (43, 44, 45) | 1.515711 | 3 | 0.000113 | 1.251063e-07 | 1.251063e-07 | 0.001111 |
55 | 0.000893 | 0.000212 | 0.000646 | 0.001477 | 50 | (2, 3, 1, 0) | (3, 244, 244, 1) | 8.339926 | 4 | 0.000893 | -2.410649e-07 | 2.410649e-07 | -0.000270 |
26 | 0.000077 | 0.000008 | 0.000069 | 0.000101 | 50 | (0, 2, 1) | (43, 44, 45) | 1.032759 | 3 | 0.000077 | 4.172780e-07 | 4.172780e-07 | 0.005440 |
39 | 0.000126 | 0.000017 | 0.000107 | 0.000185 | 50 | (2, 0, 1, 3) | (3, 244, 244, 1) | 1.180996 | 4 | 0.000115 | -1.179187e-05 | 1.179187e-05 | -0.093246 |
66 | 0.000195 | 0.000019 | 0.000163 | 0.000258 | 50 | (0, 3, 1, 2) | (1, 244, 244, 3) | 1.598770 | 4 | 0.000210 | 1.510728e-05 | 1.510728e-05 | 0.077417 |
50 | 0.000692 | 0.000136 | 0.000529 | 0.001021 | 50 | (0, 3, 2, 1) | (3, 244, 244, 1) | 6.460759 | 4 | 0.000709 | 1.714180e-05 | 1.714180e-05 | 0.024778 |
76 | 0.000824 | 0.000288 | 0.000515 | 0.001879 | 50 | (1, 3, 2, 0) | (1, 244, 244, 3) | 6.752663 | 4 | 0.000843 | 1.902846e-05 | 1.902846e-05 | 0.023087 |
54 | 0.000818 | 0.000278 | 0.000625 | 0.001522 | 50 | (2, 1, 3, 0) | (3, 244, 244, 1) | 7.634646 | 4 | 0.000843 | 2.572773e-05 | 2.572773e-05 | 0.031471 |
1 | 0.000048 | 0.000003 | 0.000045 | 0.000058 | 50 | (0, 1, 3, 2) | (12, 13, 15, 18) | 0.820821 | 4 | 0.000000 | -4.837787e-05 | 4.837787e-05 | -1.000000 |
2 | 0.000049 | 0.000003 | 0.000045 | 0.000062 | 50 | (3, 0, 1, 2) | (12, 13, 15, 18) | 0.823070 | 4 | 0.000000 | -4.851040e-05 | 4.851040e-05 | -1.000000 |
120 | 0.005918 | 0.001085 | 0.004598 | 0.008312 | 15 | (1, 0, 3, 2) | (3, 244, 244, 6) | 1.597357 | 4 | 0.008259 | 2.341673e-03 | 2.341673e-03 | 0.395716 |
128 | 0.010677 | 0.001457 | 0.008504 | 0.014070 | 15 | (2, 3, 1, 0) | (3, 244, 244, 6) | 2.882191 | 4 | 0.008132 | -2.545011e-03 | 2.545011e-03 | -0.238356 |
121 | 0.006106 | 0.000556 | 0.005619 | 0.007305 | 15 | (2, 0, 3, 1) | (3, 244, 244, 6) | 1.648325 | 4 | 0.008700 | 2.593662e-03 | 2.593662e-03 | 0.424746 |
118 | 0.003780 | 0.000882 | 0.002701 | 0.005402 | 15 | (3, 0, 1, 2) | (3, 244, 244, 6) | 1.020432 | 4 | 0.006488 | 2.707333e-03 | 2.707333e-03 | 0.716171 |
115 | 0.003653 | 0.000874 | 0.002567 | 0.005244 | 15 | (1, 2, 3, 0) | (3, 244, 244, 6) | 0.986071 | 4 | 0.006488 | 2.834624e-03 | 2.834624e-03 | 0.775972 |
129 | 0.012421 | 0.003052 | 0.007818 | 0.018106 | 15 | (3, 2, 1, 0) | (3, 244, 244, 6) | 3.352770 | 4 | 0.009386 | -3.034652e-03 | 3.034652e-03 | -0.244323 |
119 | 0.004938 | 0.000367 | 0.004532 | 0.005844 | 15 | (1, 3, 0, 2) | (3, 244, 244, 6) | 1.333061 | 4 | 0.008700 | 3.761588e-03 | 3.761588e-03 | 0.761694 |
127 | 0.010339 | 0.002789 | 0.005878 | 0.018301 | 15 | (0, 3, 2, 1) | (3, 244, 244, 6) | 2.790823 | 4 | 0.005271 | -5.068171e-03 | 5.068171e-03 | -0.490205 |
130 | 0.013432 | 0.004496 | 0.006536 | 0.021250 | 15 | (3, 2, 0, 1) | (3, 244, 244, 6) | 3.625680 | 4 | 0.008132 | -5.299336e-03 | 5.299336e-03 | -0.394540 |
131 | 0.014579 | 0.004026 | 0.007144 | 0.020739 | 15 | (3, 0, 2, 1) | (3, 244, 244, 6) | 3.935483 | 4 | 0.008259 | -6.320138e-03 | 6.320138e-03 | -0.433499 |
All big errors are negative. The model seems to give a lower value for all big errors. These errors may be outliers, the processor was busy doing something else at that time.
s = data_err.sort_values('predict')
pandas.concat([s.head(n=10), s.tail(n=10)])
average | deviation | min_exec | max_exec | number | perm | shape | ratio | dim | predict | err | abserr | rel | |
---|---|---|---|---|---|---|---|---|---|---|---|---|---|
20 | 0.000158 | 0.000021 | 0.000127 | 0.000192 | 50 | (3, 0, 2, 1) | (12, 13, 15, 18) | 2.684876 | 4 | 0.000000 | -0.000158 | 0.000158 | -1.000000 |
42 | 0.000147 | 0.000017 | 0.000106 | 0.000175 | 50 | (0, 1, 3, 2) | (3, 244, 244, 1) | 1.369978 | 4 | 0.000000 | -0.000147 | 0.000147 | -1.000000 |
34 | 0.000151 | 0.000016 | 0.000136 | 0.000197 | 50 | (1, 2, 0) | (3, 244, 244) | 1.438446 | 3 | 0.000000 | -0.000151 | 0.000151 | -1.000000 |
33 | 0.000124 | 0.000017 | 0.000108 | 0.000171 | 50 | (2, 0, 1) | (3, 244, 244) | 1.185666 | 3 | 0.000000 | -0.000124 | 0.000124 | -1.000000 |
44 | 0.000189 | 0.000044 | 0.000142 | 0.000265 | 50 | (1, 2, 3, 0) | (3, 244, 244, 1) | 1.766905 | 4 | 0.000000 | -0.000189 | 0.000189 | -1.000000 |
27 | 0.000097 | 0.000004 | 0.000083 | 0.000110 | 50 | (2, 0, 1) | (43, 44, 45) | 1.300915 | 3 | 0.000000 | -0.000097 | 0.000097 | -1.000000 |
25 | 0.000074 | 0.000009 | 0.000065 | 0.000109 | 50 | (0, 1, 2) | (43, 44, 45) | 1.000000 | 3 | 0.000000 | -0.000074 | 0.000074 | -1.000000 |
24 | 0.000073 | 0.000009 | 0.000062 | 0.000094 | 50 | (1, 2, 0) | (43, 44, 45) | 0.985513 | 3 | 0.000000 | -0.000073 | 0.000073 | -1.000000 |
22 | 0.000214 | 0.000060 | 0.000136 | 0.000295 | 50 | (1, 0, 3, 2) | (12, 13, 15, 18) | 3.627240 | 4 | 0.000000 | -0.000214 | 0.000214 | -1.000000 |
21 | 0.000164 | 0.000045 | 0.000124 | 0.000231 | 50 | (3, 1, 2, 0) | (12, 13, 15, 18) | 2.778193 | 4 | 0.000000 | -0.000164 | 0.000164 | -1.000000 |
128 | 0.010677 | 0.001457 | 0.008504 | 0.014070 | 15 | (2, 3, 1, 0) | (3, 244, 244, 6) | 2.882191 | 4 | 0.008132 | -0.002545 | 0.002545 | -0.238356 |
130 | 0.013432 | 0.004496 | 0.006536 | 0.021250 | 15 | (3, 2, 0, 1) | (3, 244, 244, 6) | 3.625680 | 4 | 0.008132 | -0.005299 | 0.005299 | -0.394540 |
122 | 0.006722 | 0.001807 | 0.005067 | 0.011245 | 15 | (1, 3, 2, 0) | (3, 244, 244, 6) | 1.814552 | 4 | 0.008259 | 0.001537 | 0.001537 | 0.228654 |
125 | 0.007815 | 0.001757 | 0.005932 | 0.010779 | 15 | (2, 1, 3, 0) | (3, 244, 244, 6) | 2.109489 | 4 | 0.008259 | 0.000444 | 0.000444 | 0.056871 |
120 | 0.005918 | 0.001085 | 0.004598 | 0.008312 | 15 | (1, 0, 3, 2) | (3, 244, 244, 6) | 1.597357 | 4 | 0.008259 | 0.002342 | 0.002342 | 0.395716 |
123 | 0.007071 | 0.000982 | 0.005454 | 0.008559 | 15 | (3, 1, 0, 2) | (3, 244, 244, 6) | 1.908667 | 4 | 0.008259 | 0.001188 | 0.001188 | 0.168070 |
131 | 0.014579 | 0.004026 | 0.007144 | 0.020739 | 15 | (3, 0, 2, 1) | (3, 244, 244, 6) | 3.935483 | 4 | 0.008259 | -0.006320 | 0.006320 | -0.433499 |
121 | 0.006106 | 0.000556 | 0.005619 | 0.007305 | 15 | (2, 0, 3, 1) | (3, 244, 244, 6) | 1.648325 | 4 | 0.008700 | 0.002594 | 0.002594 | 0.424746 |
119 | 0.004938 | 0.000367 | 0.004532 | 0.005844 | 15 | (1, 3, 0, 2) | (3, 244, 244, 6) | 1.333061 | 4 | 0.008700 | 0.003762 | 0.003762 | 0.761694 |
129 | 0.012421 | 0.003052 | 0.007818 | 0.018106 | 15 | (3, 2, 1, 0) | (3, 244, 244, 6) | 3.352770 | 4 | 0.009386 | -0.003035 | 0.003035 | -0.244323 |
Correlation between predictors#
cc = DataFrame(dict([(c['name'], numpy.maximum(c['model'].predict(X), 0)) for c in coefs]))
cc['yt'] = yt
cc
reg | med | Lasso-0.010000 | Lasso-0.100000 | Lasso-0.200000 | pos | medpos | yt | |
---|---|---|---|---|---|---|---|---|
0 | 0.298789 | 0.052436 | 0.000000 | 0.000000 | 0.000000 | 0.000000 | 0.000000 | 0.044222 |
1 | 0.000000 | 0.071575 | 0.000000 | 0.000000 | 0.000000 | 0.000000 | 0.000000 | 0.048378 |
2 | 0.000000 | 0.048393 | 0.000000 | 0.000000 | 0.000000 | 0.000000 | 0.000000 | 0.048510 |
3 | 0.000000 | 0.048393 | 0.000000 | 0.000000 | 0.000000 | 0.000000 | 0.000000 | 0.048954 |
4 | 0.248089 | 0.050781 | 0.000000 | 0.000000 | 0.000000 | 0.000000 | 0.000000 | 0.050805 |
... | ... | ... | ... | ... | ... | ... | ... | ... |
127 | 5.270700 | 4.177012 | 4.917105 | 4.615490 | 4.464429 | 4.837032 | 4.251381 | 10.338870 |
128 | 8.132342 | 7.354799 | 8.107191 | 7.646966 | 7.334861 | 7.858363 | 6.706548 | 10.677354 |
129 | 9.386005 | 8.186190 | 8.991256 | 8.082431 | 7.397300 | 8.771040 | 6.896204 | 12.420657 |
130 | 8.132342 | 7.354799 | 8.107191 | 7.646966 | 7.334861 | 7.858363 | 6.706548 | 13.431679 |
131 | 8.259236 | 7.561004 | 7.962160 | 7.605605 | 7.334861 | 7.829728 | 6.738972 | 14.579374 |
132 rows × 8 columns
cc.corr()
reg | med | Lasso-0.010000 | Lasso-0.100000 | Lasso-0.200000 | pos | medpos | yt | |
---|---|---|---|---|---|---|---|---|
reg | 1.000000 | 0.994124 | 0.996922 | 0.985715 | 0.979826 | 0.988323 | 0.980433 | 0.903528 |
med | 0.994124 | 1.000000 | 0.995863 | 0.989990 | 0.987374 | 0.990341 | 0.988401 | 0.894833 |
Lasso-0.010000 | 0.996922 | 0.995863 | 1.000000 | 0.992689 | 0.987930 | 0.994420 | 0.988358 | 0.899384 |
Lasso-0.100000 | 0.985715 | 0.989990 | 0.992689 | 1.000000 | 0.998564 | 0.998756 | 0.997985 | 0.886902 |
Lasso-0.200000 | 0.979826 | 0.987374 | 0.987930 | 0.998564 | 1.000000 | 0.995092 | 0.999385 | 0.880614 |
pos | 0.988323 | 0.990341 | 0.994420 | 0.998756 | 0.995092 | 1.000000 | 0.995169 | 0.890093 |
medpos | 0.980433 | 0.988401 | 0.988358 | 0.997985 | 0.999385 | 0.995169 | 1.000000 | 0.881208 |
yt | 0.903528 | 0.894833 | 0.899384 | 0.886902 | 0.880614 | 0.890093 | 0.881208 | 1.000000 |
Standalone predictions#
def get_coef(pipe, names):
c1 = pipe.steps[0][-1].scale_
c2 = pipe.steps[1][-1].coef_
return dict(zip(names, c2 / c1))
get_coef(coefs[-1]["model"], X.columns)
{'CST_': 0.829481835464256,
'begin': 0.0,
'dbegin': 0.0,
'dend': 0.0,
'dim': 0.08294721851224843,
'discont': 0.07025394222472751,
'edit': 0.03782977428195987,
'end': 0.0,
'end16': 0.0,
'end32': 0.0,
'ibegin16': 0.0,
'ibegin2': 0.0,
'ibegin32': 0.0,
'ibegin4': 0.0,
'ibegin64': 0.0,
'ibegin8': 0.0,
'iend16': 0.0,
'iend2': 0.0,
'iend32': 0.0,
'iend4': 0.0,
'iend64': 0.0,
'iend8': 0.0,
'middle': 3.42896339670081e-06,
'rbegin': 0.0,
'rdiscont': 0.0,
'redit': 0.0,
'rend': 0.0,
'rend16': 0.0,
'rend32': 0.0,
'rev': 0.11940214295823245,
'rmiddle': 0.0,
'rot': 0.023189032947793925,
'size': 3.021302183272755e-06}
def predict(coefs, shape, perm):
feat = compute_features(shape, perm)
res = 0
for k, v in feat.items():
res += v * coefs[k]
return res / 1000
def predict_model(model, shape, perm, names):
feat = compute_features(shape, perm)
a = numpy.zeros((1, len(names)), dtype=numpy.float64)
for i, n in enumerate(names):
a[0, i] = feat[n]
return model.predict(a) / 1000
coef = get_coef(coefs[-1]["model"], X.columns)
(predict(coef, (3, 224, 224, 6), (3, 0, 1, 2)),
predict_model(coefs[-1]["model"], (3, 224, 224, 6), (3, 0, 1, 2), X.columns))
(0.005450704959759156, array([0.0054507]))