Note
Click here to download the full example code
Benchmark and profile of operator Slice¶
This short code compares the execution of the operator Slice between numpy and onnxruntime for three configurations.
A simple example¶
import json
import numpy
from numpy.testing import assert_almost_equal
import pandas
from pandas import DataFrame
import matplotlib.pyplot as plt
from onnxruntime import InferenceSession, get_device, SessionOptions
from onnxruntime.capi._pybind_state import ( # pylint: disable=E0611
OrtValue as C_OrtValue)
from skl2onnx.common.data_types import FloatTensorType
from skl2onnx.algebra.onnx_ops import OnnxSlice, OnnxAdd, OnnxMul
from cpyquickhelper.numbers import measure_time
from tqdm import tqdm
from mlprodict.testing.experimental_c_impl.experimental_c import (
code_optimisation)
from mlprodict.onnxrt.ops_whole.session import OnnxWholeSession
from onnxcustom.utils.onnxruntime_helper import get_ort_device
print([code_optimisation(), get_device()])
Out:
['AVX-omp=8', 'CPU']
The functions to compare.
def build_ort_op(op_version=14, save=None, **kwargs): # opset=13, 14, ...
slices = kwargs['slices']
slice1, slice2 = slices
slice1 = slice(0, None) if slice1 is None else slice(*slice1)
slice2 = slice(0, None) if slice2 is None else slice(*slice2)
axes = []
starts = []
ends = []
for i in [0, 1]:
if slices[i] is None:
continue
axes.append(i)
starts.append(slices[i][0])
ends.append(slices[i][1])
starts = numpy.array(starts, dtype=numpy.int64)
ends = numpy.array(ends, dtype=numpy.int64)
axes = numpy.array(axes, dtype=numpy.int64)
node1 = OnnxSlice('X', starts, ends, axes, op_version=op_version)
node2 = OnnxAdd(node1, numpy.array([1], dtype=numpy.float32),
op_version=op_version)
node3 = OnnxSlice(node2, starts, ends, axes,
op_version=op_version)
node4 = OnnxMul(node3, numpy.array([2], dtype=numpy.float32),
op_version=op_version, output_names=['Y'])
onx = node4.to_onnx(inputs=[('X', FloatTensorType([None, None]))],
target_opset=op_version)
sess = InferenceSession(onx.SerializeToString(),
providers=["CPUExecutionProvider"])
if save is not None:
with open(save, "wb") as f:
f.write(onx.SerializeToString())
def npy_fct(x):
return ((x[slice1, slice2] + 1)[slice1, slice2] * 2).copy()
rnd = numpy.random.randn(10, 10).astype(numpy.float32)
expected = npy_fct(rnd)
got = sess.run(None, {'X': rnd})[0]
try:
assert_almost_equal(expected, got)
except AssertionError as e:
raise AssertionError(
"kwargs=%r slice1=%r slice2=%r shapes=%r ? %r "
"(x[slice1, slice2].shape)=%r" % (
kwargs, slice1, slice2, expected.shape,
got.shape, rnd[slice1, slice2].shape)) from e
if get_device().upper() == 'GPU':
sessg = InferenceSession(onx.SerializeToString(),
providers=["CUDAExecutionProvider"])
io_binding = sessg.io_binding()._iobinding
device = get_ort_device('cuda:0')
def run_gpu(x):
io_binding.bind_input(
'X', device, numpy.float32, x.shape(), x.data_ptr())
io_binding.bind_output('Y', device)
return sessg._sess.run_with_iobinding(io_binding, None)
return onx, lambda x: sess.run(None, {'X': x}), npy_fct, run_gpu
else:
return onx, lambda x: sess.run(None, {'X': x}), npy_fct, None
The benchmark.
def loop_fct(fct, xs):
for x in xs:
fct(x)
def benchmark_op(repeat=10, number=10, name="Slice", shape_slice_fct=None,
save=None, opset=14, repeat_profile=1500, verbose=1):
if verbose:
print("[benchmark_op] start repeat=%d number=%d repeat_profile=%d"
" opset=%d." % (repeat, number, repeat_profile, opset))
res = []
for dim in tqdm([8, 16, 32, 64, 100, 128, 200,
256, 400, 512, 600, 784, 800,
1000, 1024, 1200]):
shape, slices = shape_slice_fct(dim)
onx, ort_fct, npy_fct, ort_fct_gpu = build_ort_op(
save=save, op_version=opset, slices=slices)
n_arrays = 20
if dim >= 512:
n_arrays = 10
xs = [numpy.random.rand(*shape).astype(numpy.float32)
for _ in range(n_arrays)]
info = dict(shape=shape)
ctx = dict(xs=xs, loop_fct=loop_fct)
# numpy
ctx['fct'] = npy_fct
obs = measure_time(
lambda: loop_fct(npy_fct, xs),
div_by_number=True, context=ctx, repeat=repeat, number=number)
obs['dim'] = dim
obs['fct'] = 'numpy'
obs['shape'] = ",".join(map(str, shape))
obs['slices'] = str(slices)
obs.update(info)
res.append(obs)
# onnxruntime
ctx['fct'] = ort_fct
obs = measure_time(
lambda: loop_fct(ort_fct, xs),
div_by_number=True, context=ctx, repeat=repeat, number=number)
obs['dim'] = dim
obs['fct'] = 'ort'
obs['shape'] = ",".join(map(str, shape))
obs['slices'] = str(slices)
obs.update(info)
res.append(obs)
if ort_fct_gpu is not None:
# onnxruntime
dev = get_ort_device('cuda:0')
ctx['xs'] = [
C_OrtValue.ortvalue_from_numpy(x, dev)
for x in xs]
ctx['fct'] = ort_fct_gpu
obs = measure_time(
lambda: loop_fct(ort_fct_gpu, ctx['xs']),
div_by_number=True, context=ctx, repeat=repeat, number=number)
obs['dim'] = dim
obs['fct'] = 'ort_gpu'
obs['shape'] = ",".join(map(str, shape))
obs['slices'] = str(slices)
obs.update(info)
res.append(obs)
# profiling CPU
if verbose:
print("[benchmark_op] done.")
print("[benchmark_op] profile CPU.")
so = SessionOptions()
so.enable_profiling = True
sess = InferenceSession(onx.SerializeToString(), so,
providers=["CPUExecutionProvider"])
for i in range(0, repeat_profile):
sess.run(None, {'X': xs[-1]}, )
prof = sess.end_profiling()
with open(prof, "r") as f:
js = json.load(f)
dfprof = DataFrame(OnnxWholeSession.process_profiling(js))
dfprof['shape'] = ",".join(map(str, shape))
dfprof['slices'] = str(slices)
if verbose:
print("[benchmark_op] done.")
# profiling CPU
if ort_fct_gpu is not None:
if verbose:
print("[benchmark_op] profile GPU.")
so = SessionOptions()
so.enable_profiling = True
sess = InferenceSession(onx.SerializeToString(), so,
providers=["CUDAExecutionProvider"])
io_binding = sess.io_binding()._iobinding
device = get_ort_device('cpu')
for i in range(0, repeat_profile):
x = ctx['xs'][-1]
io_binding.bind_input(
'X', device, numpy.float32, x.shape(), x.data_ptr())
io_binding.bind_output('Y', device)
sess._sess.run_with_iobinding(io_binding, None)
prof = sess.end_profiling()
with open(prof, "r") as f:
js = json.load(f)
dfprofgpu = DataFrame(OnnxWholeSession.process_profiling(js))
dfprofgpu['shape'] = ",".join(map(str, shape))
dfprofgpu['slices'] = str(slices)
if verbose:
print("[benchmark_op] profile done.")
else:
dfprofgpu = None
# Dataframes
shape_name = str(shape).replace(str(dim), "N")
df = pandas.DataFrame(res)
piv = df.pivot('shape', 'fct', 'average')
rs = piv.copy()
for c in ['numpy', 'ort', 'ort_gpu']:
if c in rs.columns:
rs["numpy/%s" % c] = rs['numpy'] / rs[c]
rs = rs[[c for c in rs.columns if "/numpy" not in c]].copy()
# Graphs.
fig, ax = plt.subplots(1, 2, figsize=(12, 4))
piv.plot(logx=True, logy=True, ax=ax[0],
title="%s benchmark\n%r"
" lower better" % (name, shape_name))
ax[0].legend(prop={"size": 9})
rs.plot(logx=True, logy=True, ax=ax[1],
title="%s Speedup, baseline=numpy\n%r"
" higher better" % (name, shape_name))
ax[1].plot([min(rs.index), max(rs.index)], [0.5, 0.5], 'g--')
ax[1].plot([min(rs.index), max(rs.index)], [2., 2.], 'g--')
ax[1].legend(prop={"size": 9})
return dfprof, dfprofgpu, df, rs, ax
The results.
nth = int(code_optimisation().split('=')[1])
cols_profile = ["shape", "slices", "args_op_name", 'args_provider']
shape = (100, N) - slice = [1:-1], :¶
dfs = []
dfprof, dfprofgpu, df, piv, ax = benchmark_op(
shape_slice_fct=lambda dim: ((256, dim), ((1, -1), None)),
save="bslice.onnx", number=nth * 4, repeat=8, repeat_profile=100 * nth)
dfs.append(df)
piv2 = df.pivot("fct", "shape", "average")
print("slices = [1:-1], :")
print(piv.to_markdown())
print(dfprof.drop(['pid', 'tid', 'ts'], axis=1).groupby(
cols_profile).sum().to_markdown())
if dfprofgpu is not None:
print(dfprofgpu.drop(['pid', 'tid'], axis=1).groupby(
cols_profile).sum().to_markdown())
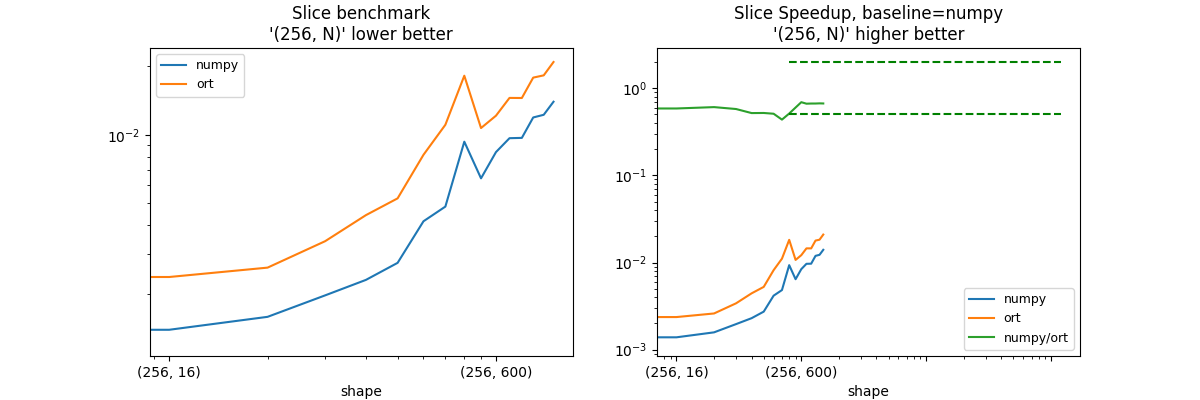
Out:
[benchmark_op] start repeat=8 number=32 repeat_profile=800 opset=14.
0%| | 0/16 [00:00<?, ?it/s]
6%|6 | 1/16 [00:00<00:13, 1.12it/s]
12%|#2 | 2/16 [00:01<00:13, 1.05it/s]
19%|#8 | 3/16 [00:02<00:13, 1.02s/it]
25%|##5 | 4/16 [00:04<00:14, 1.18s/it]
31%|###1 | 5/16 [00:06<00:15, 1.39s/it]
38%|###7 | 6/16 [00:08<00:16, 1.63s/it]
44%|####3 | 7/16 [00:11<00:19, 2.15s/it]
50%|##### | 8/16 [00:15<00:22, 2.79s/it]
56%|#####6 | 9/16 [00:22<00:29, 4.15s/it]
62%|######2 | 10/16 [00:27<00:25, 4.25s/it]
69%|######8 | 11/16 [00:32<00:22, 4.58s/it]
75%|#######5 | 12/16 [00:38<00:20, 5.10s/it]
81%|########1 | 13/16 [00:45<00:16, 5.47s/it]
88%|########7 | 14/16 [00:52<00:12, 6.15s/it]
94%|#########3| 15/16 [01:00<00:06, 6.69s/it]
100%|##########| 16/16 [01:09<00:00, 7.41s/it]
100%|##########| 16/16 [01:09<00:00, 4.37s/it]
[benchmark_op] done.
[benchmark_op] profile CPU.
[benchmark_op] done.
slices = [1:-1], :
| shape | numpy | ort | numpy/ort |
|:------------|-----------:|-----------:|------------:|
| (256, 8) | 0.00122474 | 0.00213213 | 0.57442 |
| (256, 16) | 0.00138635 | 0.00236698 | 0.585704 |
| (256, 32) | 0.00158061 | 0.00260299 | 0.607228 |
| (256, 64) | 0.00196467 | 0.00340243 | 0.577431 |
| (256, 100) | 0.00229879 | 0.00443202 | 0.518679 |
| (256, 128) | 0.00273199 | 0.00525296 | 0.520086 |
| (256, 200) | 0.00416482 | 0.00816956 | 0.509797 |
| (256, 256) | 0.00483228 | 0.011073 | 0.436402 |
| (256, 400) | 0.00932988 | 0.0182123 | 0.512283 |
| (256, 512) | 0.00643529 | 0.0107145 | 0.600618 |
| (256, 600) | 0.00839677 | 0.0121431 | 0.691486 |
| (256, 784) | 0.00966727 | 0.0145427 | 0.664752 |
| (256, 800) | 0.0096974 | 0.0145289 | 0.667455 |
| (256, 1000) | 0.0119276 | 0.0178634 | 0.66771 |
| (256, 1024) | 0.0122553 | 0.0182789 | 0.670461 |
| (256, 1200) | 0.0139913 | 0.0209278 | 0.668551 |
| | dur |
|:-----------------------------------------------------------------|-------:|
| ('256,1200', '((1, -1), None)', 'Add', 'CPUExecutionProvider') | 227335 |
| ('256,1200', '((1, -1), None)', 'Mul', 'CPUExecutionProvider') | 223940 |
| ('256,1200', '((1, -1), None)', 'Slice', 'CPUExecutionProvider') | 942561 |
shape = (100, N) - slice = :, [1:-1]¶
dfs = []
dfprof, dfprofgpu, df, piv, ax = benchmark_op(
shape_slice_fct=lambda dim: ((256, dim), (None, (1, -1))),
save="bslice.onnx", number=nth * 4, repeat=8, repeat_profile=100 * nth)
dfs.append(df)
piv2 = df.pivot("fct", "shape", "average")
print("slices = :, [1:-1]")
print(piv.to_markdown())
print(dfprof.drop(['pid', 'tid', 'ts'], axis=1).groupby(
cols_profile).sum().to_markdown())
if dfprofgpu is not None:
print(dfprofgpu.drop(['pid', 'tid'], axis=1).groupby(
cols_profile).sum().to_markdown())
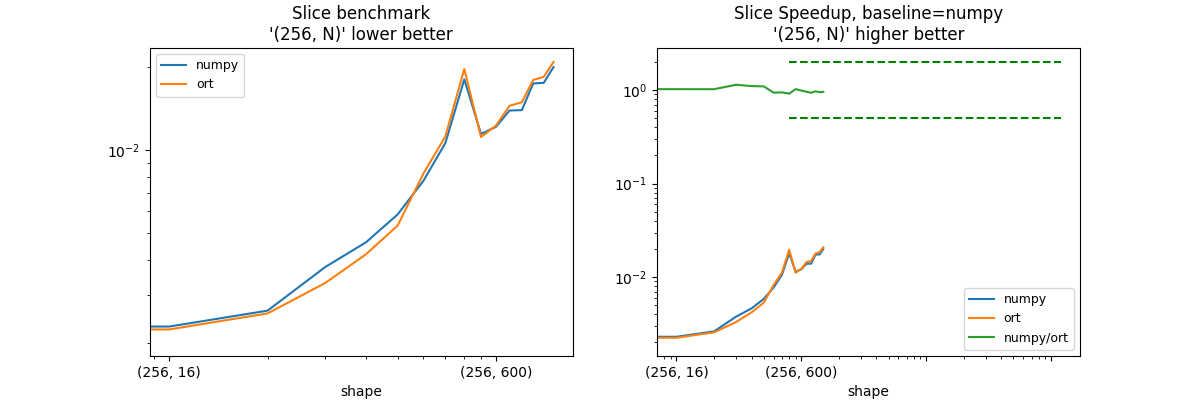
Out:
[benchmark_op] start repeat=8 number=32 repeat_profile=800 opset=14.
0%| | 0/16 [00:00<?, ?it/s]
6%|6 | 1/16 [00:01<00:16, 1.08s/it]
12%|#2 | 2/16 [00:02<00:16, 1.15s/it]
19%|#8 | 3/16 [00:03<00:16, 1.25s/it]
25%|##5 | 4/16 [00:05<00:17, 1.49s/it]
31%|###1 | 5/16 [00:07<00:19, 1.78s/it]
38%|###7 | 6/16 [00:10<00:21, 2.17s/it]
44%|####3 | 7/16 [00:14<00:25, 2.82s/it]
50%|##### | 8/16 [00:20<00:29, 3.72s/it]
56%|#####6 | 9/16 [00:30<00:39, 5.60s/it]
62%|######2 | 10/16 [00:36<00:34, 5.68s/it]
69%|######8 | 11/16 [00:42<00:29, 5.88s/it]
75%|#######5 | 12/16 [00:49<00:25, 6.33s/it]
81%|########1 | 13/16 [00:57<00:20, 6.67s/it]
88%|########7 | 14/16 [01:06<00:14, 7.42s/it]
94%|#########3| 15/16 [01:15<00:07, 7.99s/it]
100%|##########| 16/16 [01:26<00:00, 8.76s/it]
100%|##########| 16/16 [01:26<00:00, 5.39s/it]
[benchmark_op] done.
[benchmark_op] profile CPU.
[benchmark_op] done.
slices = :, [1:-1]
| shape | numpy | ort | numpy/ort |
|:------------|-----------:|-----------:|------------:|
| (256, 8) | 0.00202208 | 0.00208558 | 0.969553 |
| (256, 16) | 0.00229975 | 0.00224515 | 1.02432 |
| (256, 32) | 0.00262508 | 0.00256523 | 1.02333 |
| (256, 64) | 0.00377195 | 0.00330289 | 1.14201 |
| (256, 100) | 0.0046357 | 0.00419682 | 1.10457 |
| (256, 128) | 0.00584651 | 0.00532873 | 1.09717 |
| (256, 200) | 0.00774097 | 0.00822542 | 0.941103 |
| (256, 256) | 0.0105949 | 0.0111949 | 0.946404 |
| (256, 400) | 0.0180014 | 0.0196297 | 0.91705 |
| (256, 512) | 0.0114267 | 0.0111348 | 1.02622 |
| (256, 600) | 0.0121301 | 0.0122574 | 0.989617 |
| (256, 784) | 0.0138878 | 0.0144415 | 0.961664 |
| (256, 800) | 0.0139389 | 0.0148834 | 0.936539 |
| (256, 1000) | 0.0174043 | 0.0179164 | 0.971417 |
| (256, 1024) | 0.017487 | 0.018385 | 0.951155 |
| (256, 1200) | 0.019937 | 0.0208039 | 0.958329 |
| | dur |
|:-----------------------------------------------------------------|-------:|
| ('256,1200', '(None, (1, -1))', 'Add', 'CPUExecutionProvider') | 228597 |
| ('256,1200', '(None, (1, -1))', 'Mul', 'CPUExecutionProvider') | 237380 |
| ('256,1200', '(None, (1, -1))', 'Slice', 'CPUExecutionProvider') | 932484 |
shape = (100, N) - slice = [1:-1], [1:-1]¶
dfs = []
dfprof, dfprofgpu, df, piv, ax = benchmark_op(
shape_slice_fct=lambda dim: ((256, dim), ((1, -1), (1, -1))),
save="bslice.onnx", number=nth * 4, repeat=8, repeat_profile=100 * nth)
dfs.append(df)
piv2 = df.pivot("fct", "shape", "average")
print("slices = [1:-1], [1:-1]")
print(piv.to_markdown())
print(dfprof.drop(['pid', 'tid', 'ts'], axis=1).groupby(
cols_profile).sum().to_markdown())
if dfprofgpu is not None:
print(dfprofgpu.drop(['pid', 'tid'], axis=1).groupby(
cols_profile).sum().to_markdown())
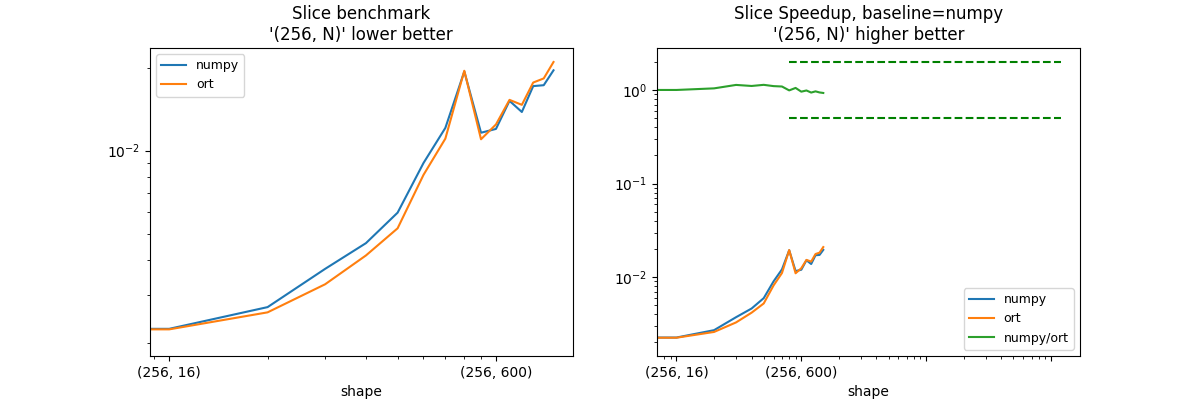
Out:
[benchmark_op] start repeat=8 number=32 repeat_profile=800 opset=14.
0%| | 0/16 [00:00<?, ?it/s]
6%|6 | 1/16 [00:01<00:16, 1.08s/it]
12%|#2 | 2/16 [00:02<00:15, 1.14s/it]
19%|#8 | 3/16 [00:04<00:18, 1.42s/it]
25%|##5 | 4/16 [00:05<00:18, 1.58s/it]
31%|###1 | 5/16 [00:08<00:20, 1.84s/it]
38%|###7 | 6/16 [00:11<00:22, 2.20s/it]
44%|####3 | 7/16 [00:15<00:26, 2.94s/it]
50%|##### | 8/16 [00:21<00:31, 3.91s/it]
56%|#####6 | 9/16 [00:31<00:40, 5.83s/it]
62%|######2 | 10/16 [00:37<00:35, 5.84s/it]
69%|######8 | 11/16 [00:43<00:29, 5.99s/it]
75%|#######5 | 12/16 [00:51<00:26, 6.57s/it]
81%|########1 | 13/16 [00:59<00:20, 6.82s/it]
88%|########7 | 14/16 [01:08<00:14, 7.48s/it]
94%|#########3| 15/16 [01:17<00:08, 8.00s/it]
100%|##########| 16/16 [01:27<00:00, 8.76s/it]
100%|##########| 16/16 [01:27<00:00, 5.49s/it]
[benchmark_op] done.
[benchmark_op] profile CPU.
[benchmark_op] done.
slices = [1:-1], [1:-1]
| shape | numpy | ort | numpy/ort |
|:------------|-----------:|-----------:|------------:|
| (256, 8) | 0.00202314 | 0.00207122 | 0.976791 |
| (256, 16) | 0.00225834 | 0.00224831 | 1.00446 |
| (256, 32) | 0.00270728 | 0.00259146 | 1.04469 |
| (256, 64) | 0.00372587 | 0.00327614 | 1.13728 |
| (256, 100) | 0.00461852 | 0.00416746 | 1.10823 |
| (256, 128) | 0.0059576 | 0.00522772 | 1.13962 |
| (256, 200) | 0.00901611 | 0.00816554 | 1.10417 |
| (256, 256) | 0.0120908 | 0.0110545 | 1.09375 |
| (256, 400) | 0.0193612 | 0.019482 | 0.993802 |
| (256, 512) | 0.0116121 | 0.010995 | 1.05613 |
| (256, 600) | 0.0119881 | 0.0124442 | 0.963347 |
| (256, 784) | 0.0151558 | 0.0152803 | 0.991856 |
| (256, 800) | 0.0138089 | 0.0146576 | 0.942097 |
| (256, 1000) | 0.0171397 | 0.0176591 | 0.970589 |
| (256, 1024) | 0.017269 | 0.0182734 | 0.945036 |
| (256, 1200) | 0.0195565 | 0.020961 | 0.932994 |
| | dur |
|:--------------------------------------------------------------------|-------:|
| ('256,1200', '((1, -1), (1, -1))', 'Add', 'CPUExecutionProvider') | 241762 |
| ('256,1200', '((1, -1), (1, -1))', 'Mul', 'CPUExecutionProvider') | 233176 |
| ('256,1200', '((1, -1), (1, -1))', 'Slice', 'CPUExecutionProvider') | 914787 |
Total running time of the script: ( 4 minutes 18.669 seconds)